By: Ian Geertsen
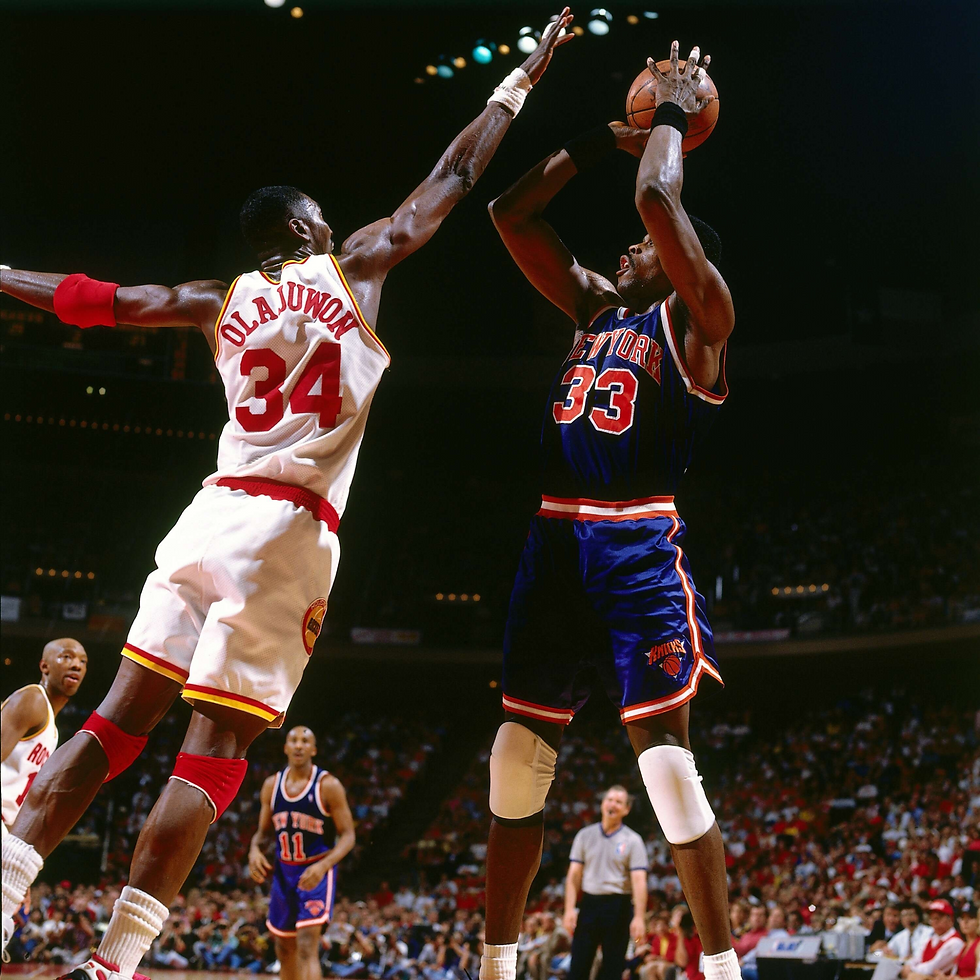
Within the last decade, the term ‘gravity’ has entered the basketball lexicon as a means of describing the noticeable and substantial impact that players can have on offense without even touching the ball. Inspired by the play of today’s perimeter superstars—chief among them being Golden State’s Steph Curry—the term ‘gravity’ refers to how a player’s threat to score, and more specifically threat to shoot, can alter the structure and attention of opposing defenses, leading to the creation of opportunities for teammates to take advantage of. Curry is the poster child of shooting gravity because his exceptional marksmanship, limitless range, lighting fast release, and constant off-ball movement make him a threat from anywhere on the court and nearly impossible to guard. This means that Curry often commands the attention not only of the player guarding him, but of other defensive players on the court as well. When multiple defenders are committing their attention—and often time their defensive positioning—to one offensive player, and that player doesn’t even have the ball, it's easy to see how a significant advantage can be created for the offensive team by our shooter’s gravitational pull alone.
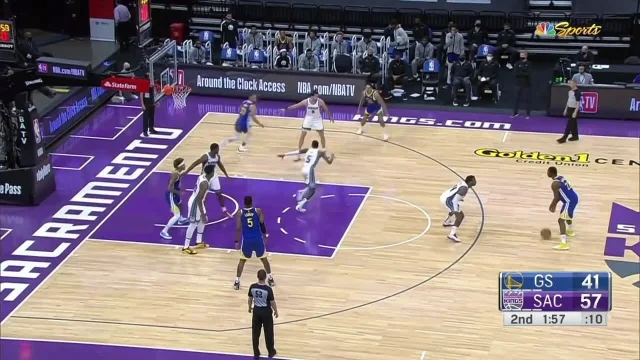
This image perfectly showcases Curry’s impact on the game without the ball; four Kings defenders have clearly shifted their attention to Curry and three are looking directly at him. Only one player seems not to be tracking Curry, the defender guarding the ball—none of the other defenders seem that interested in where the ball is, they’re too preoccupied with where Curry is.
While I could have used this piece to talk all about shooting gravity, this has been done before. This concept actually inspired me to write about what the equivalent of offensive gravity would be on the other end of the court: defensive deterrence. If the greatest offensive players in the game can have a substantial impact on the game while the ball isn’t even in their hands, I reasoned that the same must be said of the game’s best defensive players while they aren’t guarding the ball. In this case though, instead of having the ‘pulling’ effect of gravity through drawing defenders in towards them, great defenders should have a ‘repelling’ effect by pushing offensive players away. Where should they be pushed away from, you might ask? From the spot that has always been and will always be the most dangerous spot on the floor: the rim.
If Steph Curry is the model archetype for offensive gravity, the same must be said for Rudy Gobert on the defensive end. A three-time defensive player of the year and counting, Gobert’s defensive IQ, athleticism, and ridiculous length combine to create the best defensive center in the league, and one of the best—if not the best—defensive players of this generation (Draymond Green is typing…). Despite some hefty Gobert slander following Utah’s early exit in the 2020-2021 playoffs, most fans and all players recognize the dominance Gobert brings on the defensive end; so shouldn’t that translate to how opposing players play offense against him? More specifically, shouldn’t Gobert’s mere presence under the rim affect an opposing offenses’ shot selection without him needing to block a single shot, much as Curry’s presence affects the makeup of opposing defenses without him needing to swish a single three? This is the question that I hope to answer in this paper, not just for Gobert but for the league at large.

The Metric
Before getting into the metric, there are a few concepts I want to address first. Going back to offensive gravity, gravity is generated first and foremost by the offensive skill of shooting, and more specifically long-range shooting. This means that there can be great offensive players who, if they do not have a good three point shot, may exhibit low offensive gravity despite their high offensive impact. Likewise, defensive deterrence is all about repelling offensive players away from the rim, where shooting percentages and foul-drawing rates are often the highest. This means that defensive deterrence is exhibited mainly by rim-protecting bigs; other players of a different defensive archetype may have a strong defensive impact but exhibit weak defensive deterrence simply because that is not their role in the defense—more on this later.
While I would’ve loved to run every NBA player through this analysis just for the sake of it, I had to manually pull most of the data, making that unfeasible. Additionally, as mentioned above, this analysis is most conducive to a specific kind of defensive player, being a rim-protector. Because of this, I decided to limit my analysis to a smaller sample—consisting of 63 players—that I felt fit the archetype I was looking for. I made sure to choose players who I felt were both very good protectors as well as very bad protectors, as this would allow me to test the accuracy of my metric. Additionally, I also wanted to add some players other than just rim-protecting bigs to see how they would fare in the metric; if I’m looking at a more traditional defensive wing, for instance, he should score worse in defensive deterrence than he would relative to an all-encompassing defensive metric, because my metric is attempting to quantify a skill that is not his specialty. I also included data from two players—Nicola Vučević and Kelly Olynyk—who were traded midway through the 2020-20201 season; analyzing these players before and after their trades should help us better understand how a player’s context affects their defensive deterrence rating.
The metric I developed to try and quantify defensive deterrence was limited to what publicly-available data I could find. Most of the data I used came directly from the NBA, and can be described by these five categories: opponents shots from less than five feet, opponents shots from the restricted area, opponents shots from the paint (non RA), opponents shots from mid-range, and opponents free throws attempted. Seems simple enough, right? The basic theory that I carried while determining this metric was that when players exhibiting higher defensive deterrence are on the floor, the opposing offense should shoot fewer higher-percentage two point shots (i.e. fewer shots from less than five feet, the restricted area, and the paint) and more lower-percentage two point shots (i.e. more mid-range shots). A good defense will prevent the opposing team from shooting shots closer to the rim because the closer you get to the hoop the more points you are likely to produce; over the 2020-2021 season, NBA players shot an average of 64.17% in the restricted area, 42.64% in the paint (non restricted area), and 41.39% from mid-range. I also considered how the presence of these players would affect the opposing team’s three point attempt rate, but considering that three pointers themselves can be highly efficient shots I figured that was a can of worms for another day. Since the presence of a high impact defensive deterrer should result in less drives to the basket, I also theorized that the presence of these players should result in the opposing offense attempting less free throws, as shots near and around the rim have a much higher foul-drawing rate than jumpers from farther away from the hoop. Now that we have this established, onto the tricky part.
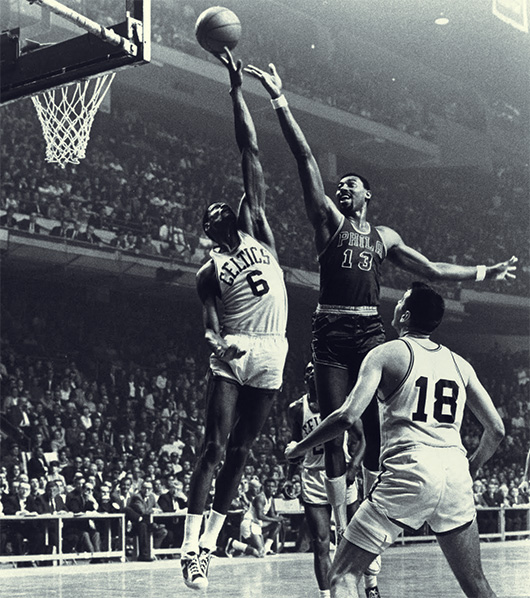
Bill Russell (left) blocks Wilt Chamberlain’s (center) attempt at the rim, showcasing why he is still considered one of the great defenders in NBA history.
Player vs Team Comparisons
NBA.com gives per game data on each of the above five categories of opponent shooting for teams and for players. That data by itself isn’t all that useful, but what would be useful is knowing opponents’ shooting patterns when one player is on the court vs when that one player is off the court. Using data on the player’s opponent shooting attempts, team’s opponent shooting attempts, and the team and player’s overall minutes played I can calculate the on/off values for each of these five categories for each player in my sample. For instance, let’s say that Clint Capella allows an average of 17.7 opponent shots in the restricted area per game when he is on the floor, and that the Hawks allow an average of 27.9 opponent shots in the restricted area per game as a team. These numbers aren’t exactly comparable as Capella averages 30.1 minutes played per game, while the Hawks average 48 minutes and change—because of overtime—minutes played per game (because overtime accounts for so few minutes played compared to the rest of the season, we can assume that every team averages 48 minutes played without having a discernible effect on our data). If we convert these numbers into per minute values, we can now see that Capella allows an average of 0.588 opponent shots in the restricted area per minute, while the Hawks allow an average of 0.581 opponent shots in the restricted area per minute. This, however, still does not tell us how opponents shoot against the Hawks when Capella is on the floor compared to when he is off the floor. To find that, we need to calculate shots attempted in the restricted area with Capella off the floor; to get this information, we need to know how many minutes Capella played during the season (1,898 minutes played) and how many minutes the Atlanta Hawks played during the season (3,481 minutes played). The calculations for how many shots in the restricted area were attempted against the Hawks per minute when Capella was off the floor are as follows:
(team mins/player mins off court)*(team shots RA * player shots RA(player mins on/team mins))
Doing this calculation tells us that the Atlanta Hawks allowed 0.573 shots in the restricted area per minute when Capella was off the floor. Now, we can compare how offenses choose their shots with Capella on the floor vs off the floor:
(player shots RA) / [(team mins/player mins off court)*(team shots RA * player shots RA(player mins on/team mins))]
Atlanta’s opponent shots in the RA with Capella on the floor (0.588) divided by Atlanta’s opponent shots in the RA with Capella off the floor (0.573) gives us a ratio of 1.026. When this ratio is higher than one, we can see that the team allowed more shots from a given area with a player on the court compared to off the court. Since shots in the restricted area are generally favorable ones for an offense, the lower the ratio is the better. This actually brings up an important point about the metric as a whole, which is that lower values are better and higher values are worse.
Capella might not have performed ideally in this category, but what about the rest of the sample? If we isolate this one part of the metric we can see that our five top performers from our sample are Tristan Thompson, Rudy Gobert, Aron Baynes, Myles Turner, and Paul Millsap. Here we see some names we would expect and some others we might not, but this is only one small piece of the puzzle.
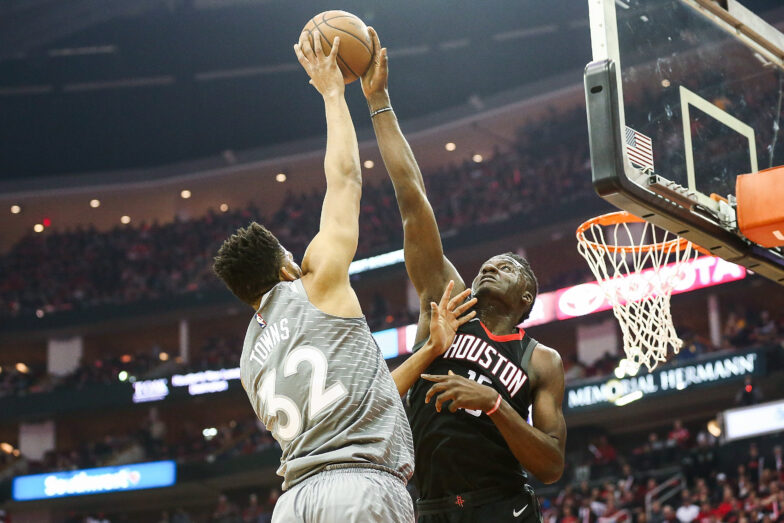
Player vs League Comparisons
The analysis we’ve done so far has solely focused on generating on-off data for individual players. While this data is very important and makes up the backbone of the metric, on-off data is far from perfect in that it is affected by the quality of the players around you. For instance, sharing minutes with a really good player can make your on-off numbers look better than they should, and being replaced by a very bad player can have the same effect. Since we are mostly looking at rim-protecting bigs, of which teams are likely to only have one on the floor at a time, the second effect is likely to carry a larger impact than the first. This means that players who play with especially bad replacement defensive deterrers will have their on-off numbers bolstered simply because their replacement does a bad job, which is obviously not what we want reflected in the metric. To help balance this out, we can also compare a player’s values directly to the values for the league at large. When we do this, however, we now face the problem that a player playing on a good defensive team will have their overall defensive numbers look better than league average numbers just because they are playing on a good team, a problem that was not nearly concerning when we were making within-team evaluations. To account for this difference, we can slightly punish players who play on teams that perform well in the category we are evaluating. All of this combines to create a formula for comparing player’s to league averages that looks like this:
(player shots RA / league avg. shots RA)*((league avg. shots RA / team shots RA)^0.5)
I know I’m throwing a lot at you right now, but the gist of what I’m saying is this: comparing a player to the rest of their team isn’t perfect because it will be influenced by the quality of their replacements, and comparing a player to the entire league isn’t perfect because it will be influenced by the quality of the rest of their team. To hit the sweet spot, we should use a little bit of both; but how should we weigh these two formulas respective to one another? I view the player-to-team formula as more telling of on-court impact and I believe the player-to-league comparison is messier and less accurate, so I decided to weigh the player-to-team formula as four times more important in the scheme of the metric. All in all, this is what the formula for opponent shots in the restricted area looks like:
(player shots RA) / [(team mins/player mins off court)*(team shots RA * player shots RA(player mins on/team mins))] + (0.25)*(player shots RA / league avg. shots RA)*((league avg. shots RA / team shots RA)^0.5)
Between Category Differences
So far I have been using opponent shots in the restricted area to demonstrate how I developed the metric, but that is just one of the five major categories I used in the analysis. Of the other four, opponent shots within five feet of the rim, opponent shots in the paint (non restricted area), and opponent free-throws attempted are calculated the same way because the theory behind them is the same; good defensive deterrence will cause an offense to shoot less shots from within five feet, less shots from the paint, and draw less free throws. Our final category, however, tells a different story.
A player exhibiting impactful defensive deterrence should actually cause an opposing offense to attempt more mid-range jumpers, as these shots should be less favorable looks compared to ones closer to the basket. Because of this, when making the calculations for this category we flip the ratio so that allowing more mid-range jumpers reflects positively in the metric and not negatively (as allowing less shots would for each of the other four categories). As a result, the formula for opponent mid-range shots is as follows:
[(team mins/player mins off court)*(team shots MDR * player shots MDR(player mins on/team mins))] / (player shots MDR) + (0.25)*(player shots MDR / league avg. shots MDR)*((league avg. shots MDR / team shots MDR)^0.5)
Once we have the calculations for each of the five categories done, we then have to decide how to weigh each individual category compared to the other four. This weighing is done based on how relevant I believe each category is to quantifying defensive deterrence. Because they deal with shots closest to the basket—which are the two-point shots that a defense strives to take away the most—I see the categories of opponent shots within five feet and opponent shots in the restricted area as being more indicative of defensive deterrence. Coming in just behind them are shots in the paint (non-RA). These shots are still valuable to an offense, but they are not as valuable as attempts at the rim and therefore are not as catastrophic for a defense to give up. This means that these shots in the paint should still be seen as very important, just not quite as important as closer attempts. It's worth noting that the restricted area extends out four feet, so there will be some overlap between categories; this is a good thing because it allows the data to use different perspectives, in theory creating a more rounded and less biased measurement. After shots in the paint, we then have opponent shots from the mid-range. This category is slightly more convoluted because of its inverted nature, but I believe it is still very important to the overall calculus of this metric. If a good protector reduces shots at the rim, those extra shots must be coming from somewhere. If those shots are coming from mid-range twos instead of threes, better defensive outcomes would be more likely to occur as a result, and this shot selection change would be more indicative of good interior defense and not some other factor than a shift to more three-pointer shots. Finally, we have opponent free throws attempted. Because free throws can be allotted for many reasons and fouls can be called on any shot attempt, I see this category as the overall weakest predictor of defensive deterrence. While I still found it important enough to include in the metric, I gave it the lowest relative weighting. All in all, here’s what the metric looks like now:
(opp. shots <5ft) + (opp. shots RA) + (0.9)(opp. shots paint) + (0.85)(opp. shots MDR) + (0.8)(opp. FTA)
Team Responsibility
The last adjustment we have to make to our metric is accounting for team responsibility. As you have seen, these calculations largely rely on measuring the shot selection of opposing offenses when a player is on the floor vs off the floor; despite previous adjustments we’ve already made, this means that players will be impacted by the play of the other four defenders who share the court with them at any given time. According to the metric as of now, if a center and a point guard share precisely all of their minutes they should both end up with the same defensive deterrence score for that game, although it is unlikely that they both contributed the same amount in this regard. To account for this, I added one final calculation attempting to quantify the responsibility a defender has for protecting the rim and the areas around it; if a player scores extremely well in defensive deterrence but has a low team responsibility rating, their values are likely being bolstered by the presence of another defensive player. This should be reflected in their defensive deterrence valuation accordingly.
While our previous data told us the frequency of shots from an entire offense when a player was on the floor, the data we use to calculate team responsibility looks at how many shots were defended by an individual player in certain areas on the court. More specifically, we can see the frequency that a player defends shots from within six feet of the basket and shots at the rim. If a player defends more of these shots, it can be reasoned that they play a larger role in the rim-protection of their team’s defense and therefore are having a larger relative impact on repelling offensive players than defenders who aren’t contesting many shots near the hoop. This may seem slightly counterintuitive to the rest of the analysis, as in this case defending more shots reflects positively in the metric despite the fact that these shots are being taken from close to the basket. Here is my reasoning behind this piece of the metric: offenses will always get shots off at the rim, no defense will ever be able to take that away completely. A great rim protector should defend a disproportionately large amount of these shots, as that is their role within the defense, but the presence of said rim protector should also dissuade offensive players from attacking the basket. This is what the calculation for team responsibility looks like:
(((team DFGA <6ft / player DFGA <6ft - 1) ^ 0.25) * (((team DFGA rim / player DFGA rim - 1) ^ 0.25)) ^ 0.5
Remember, positive valuations in this metric are still reflected by small numbers, so the lower the score the more relative responsibility you should have. The reason why the formula includes values being raised to the ¼ and ½ is because I want team responsibility to have a very low variance. This responsibility value will be multiplied with the previous portion of the metric to give us our overall defensive deterrence values, and I didn’t want the addition of team responsibility to cause any drastic changes to the metric's evaluations.
Finally, here is what the defensive deterrence metric looks like in its entirety:
((opp. shots <5ft) + (opp. shots RA) + (0.9)(opp. shots paint) + (0.85)(opp. shots MDR) + (0.8)(opp. FTA)) * (((team DFGA <6ft / player DFGA <6ft - 1) ^ 0.25) * (((team DFGA rim / player DFGA rim - 1) ^ 0.25)) ^ 0.5
Once these values were calculated, I normalized this part of the metric by setting the average to one, meaning the average player among my sample should not have their defensive deterrence score affected at all by team responsibility.
See Part II for the continuation.
Sources
Data: https://docs.google.com/spreadsheets/d/1kWXUFkNLD-t_DbgcVKfYnab5o18xSy6vD7Hqe7huGA/edit?usp=sharing
Sources: NBA.com, basketballreference.com, bball-index.com, espn.com.
Comments