By: Sean Gee and Praharsh Mehrotra
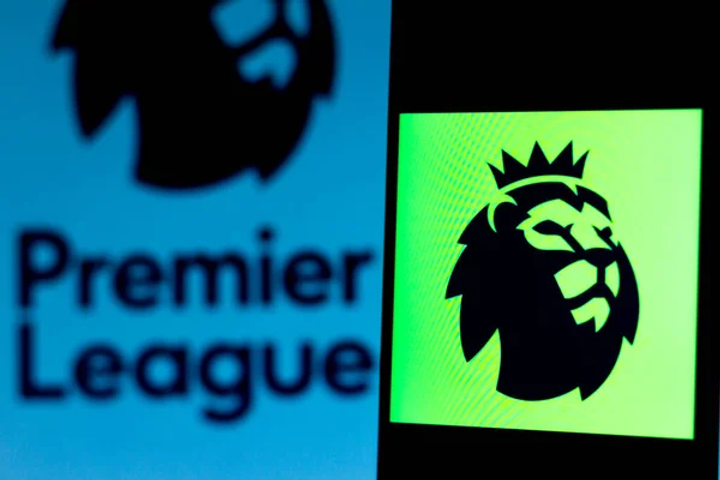
Introduction
The English Premier League (EPL) stands as one of the most competitive and financially lucrative football leagues globally, attracting top talent with record-breaking transfer fees. Clubs invest heavily in players, banking on their ability to perform and transform team dynamics. However, the burning question persists: does the money spent on transfer fees truly reflect a player's impact on the pitch?
In recent years, the EPL has witnessed a series of high-profile transfers, with clubs paying astronomical sums to acquire players they believe will secure success. While some transfers have paid dividends, others have fallen short, sparking debates about the effectiveness of transfer fees as a predictor of performance. For instance, how do players with similar market values perform when placed in different tactical systems? Can a club's investment be justified purely by individual metrics such as passes completed or minutes played?
Average Market Value
Building on this, the central research question emerges: Is the transfer amount a reliable predictor of player performance? Intuitively, one might wonder, does a higher transfer fee motivate players to excel, or does the financial burden create pressure, hindering their performance? To explore this, we focus our investigation on the English Premier League, excluding winter transfers to maintain consistency.
First, we would like to see the variety of Teams in the English Premier League and the market value per club.
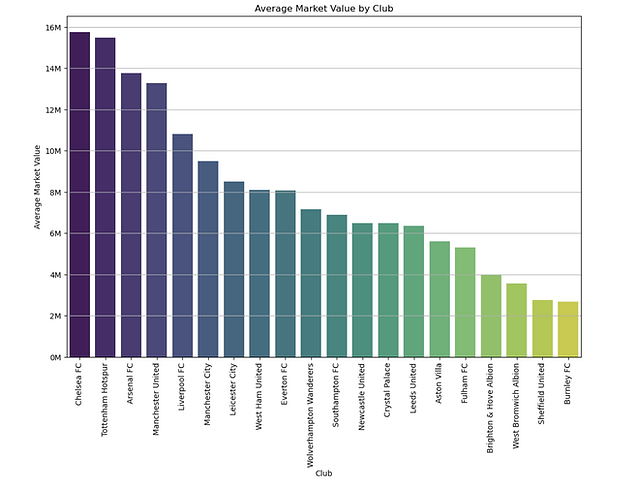
From the average market value by club, we can say that clubs like Chelsea, Arsenal, and Manchester United invest heavily in high-value players, creating pressure to justify their transfer fees. In contrast, teams like Burnley and Sheffield United adopt budget-conscious approaches, focusing on player development. This contrast raises a key question: is there a relationship between market value and transfer fees?

The relationship between a player’s market value and their transfer fee demonstrates a noticeable positive trend: players with higher market values tend to command higher transfer fees. Market value refers to an estimation of a player's worth based on their skills, age, and recent performances, as evaluated by analysts, scouts, and sports market databases. Transfer fees, on the other hand, represent the amount of money a club pays to acquire a player under contract with another team.
While this trend suggests that market value is often a guiding factor in determining transfer fees, the alignment is far from exact. In practice, transfer fees can deviate significantly from market valuations. These discrepancies arise due to a variety of external factors. For instance, a club might pay a premium for a player if they urgently need to fill a specific position or if the player has a reputation as a game-changer. Conversely, players might be acquired for less than their estimated worth due to contract expirations, injury concerns, or limited interest from other teams.
Understanding this dynamic reveals the complexity of evaluating a player’s true value. Although market value provides a structured baseline for negotiations, it does not fully account for the subjective and contextual factors that drive transfer fees. This variability adds an important layer of uncertainty to whether transfer fees can reliably predict a player’s performance on the pitch. For example, a high transfer fee might indicate expectations of elite performance, but it can also reflect external pressures such as market demand or a club’s financial strength, rather than the player’s actual ability to deliver results.
Pass Percentage Model
In this section, we will focus primarily on pass percentage data for positions other than forwards and goalkeepers. By combining data gathered from Kaggle.com, we were able to combine the player’s performances with their overlapping transfer values in order to see whether player performance can affect their overall monetary values in the current year. Goalkeepers were excluded because their performance is not closely tied to metrics like assists or passes. Meanwhile, Forwards will be looked into further in the article. Midfielders and defenders play pivotal roles in maintaining possession, dictating the tempo of the game, and executing tactical strategies. Passing accuracy and efficiency are critical metrics for these positions, reflecting not only their individual skills but also their compatibility with the team’s overall playing style.

Methodology:
Box-Cox Transformation:
To analyze pass completion percentages for midfielders and defenders, we applied the Box-Cox transformation to stabilize variance and reduce skewness.
The original data (left histogram) was irregular and skewed, while the transformed data (right histogram) became more symmetrical and closer to a normal distribution.
Q-Q Plot:
The Q-Q plot (bottom) compares the transformed pass completion data to a normal distribution.
The alignment of points along the red line confirms the transformation successfully normalized the data.
Why This Matters:
By normalizing pass completion rates, we can see that players with consistently higher pass completion rates might hold greater monetary value in the transfer market. This is promising because it suggests a potential correlation between passing accuracy and market value. However, it’s not confirmed yet. Playtime in minutes is still a key factor that could influence these rates. For example, a player who plays a shorter amount of time might complete more passes efficiently, while another player who plays longer could see their pass rate dip. So, while the data looks promising, we still need to account for playtime to get the full picture.
White’s Robust Standard Error Model

In order to account for players' time and passing, we created and used White's robust standard error model, which adjusts for any irregularities in the data, like uneven variance. In simple terms, this method ensures our results remain reliable even if the data isn't perfectly clean or evenly spread, kind of like adding an extra layer of quality control.
Using this approach, we found that passes attempted had a significant and positive impact on playing time. Specifically, for every additional pass attempted, players spent approximately 2.61 more minutes on the pitch (p < 0.001). This shows that staying active and contributing to ball movement is highly valued when determining playing time. This makes intuitive sense, players heavily involved in moving the ball contribute to their team's strategy and become integral to their style of play. A midfielder orchestrating the tempo or a defender initiating attacks from the back is more than just a player; they're a critical part of the team's tactics. The numbers back this up, showing a robust and highly significant relationship.
In contrast, market value did not have a significant impact (p = 0.230), suggesting that being an expensive player doesn’t guarantee more minutes. Instead, market value likely reflects a player's reputation or potential rather than their actual role on the team. Lastly, the pass completion percentage also lacked a significant relationship (p = 0.336). This indicates that while accuracy matters, teams seem to prioritize the volume of passes attempted, rewarding activity and involvement over perfection.
Additional Factors
Before we finished off with a final robust model that incorporates the regression analysis, we wanted to have a look at whether there were other combinations of metrics that might reveal hidden relationships. For example, we explored whether the interaction between a player’s passing attempts and their pass completion percentage influenced their minutes played.

We included an interaction term in our robust error model between a player’s passes attempted and their pass completion percentage using our regression model. An interaction term essentially checks if the combined effect of two variables creates a unique impact beyond their individual contributions. In this case, it’s like asking whether attempting more passes while maintaining high accuracy has an extra influence on playing time. However, the interaction term was not statistically significant (p = 0.517). This means that while attempting more passes and completing them accurately are valuable on their own, their combined effect doesn’t appear to add any additional impact on minutes played. Essentially, involvement (attempting passes) and accuracy contribute independently, but they don’t amplify each other when combined.
This highlights the complexity of football, coaches value players who stay active and involved, but perfection in passing doesn’t necessarily elevate their time on the pitch beyond what they already contribute.

We also looked at how the number of passes attempted and predicted minutes on the pitch influenced a player’s perceived value and role within their team. Unlike earlier in the Robust Standard Error Model, where we tested multiple factors like pass completion percentage and market value alongside passes attempted to see if minutes could correlate with the number of passes attempted, this graph focuses solely on the strong relationship between passes attempted and predicted minutes. It highlights that, while other metrics showed no significant impact, pass volume remains the key driver of playing time, emphasizing a player’s active involvement in their team’s dynamics.
The graph illustrates this relationship clearly: as passes attempted to increase, the predicted minutes on the pitch also rise steadily. This connection underscores the tactical importance of players who are consistently involved in passing sequences. These players don’t just move the ball; they drive the rhythm and strategy of the game. The interplay between their on-pitch contributions and the time they are given to make those contributions highlights their critical role in the overall team dynamic.
Final Model

In our final model, we brought together all the tools discussed and analyzed the interaction between passes attempted and market value and observed a statistically significant result (p = 0.024). This indicates that players with higher market values see an amplified benefit from attempting more passes. For example, a highly valued player attempting 50 passes is likely to experience a greater boost in playing time compared to a less expensive player attempting the same number. This suggests that technical ability (measured by passes attempted) and perceived value (market value) work together, creating a synergy that further elevates the player’s importance to the team.
This finding highlights how a player’s reputation and on-pitch involvement interact to shape their role. Teams appear more inclined to rely on high-value players who actively contribute through ball movement, reinforcing the idea that both skill and status matter. Ultimately, the combination of pass volume and market value underscores the nuanced ways clubs determine playing time, where top players are rewarded not just for their price tag but for staying consistently engaged in the game.
So what does this mean for Premier League fans? Firstly, passing as a skill emerges as a cornerstone of a player’s contribution. While goals and assists grab the headlines, it's the players who keep the ball moving who are truly vital to the team. Secondly, market value, while significant, acts more as an amplifier of other skills rather than a standalone determinant of playing time. For fans, this means looking beyond transfer fees to understand the real value of a player. For clubs, it’s a reminder that the most effective players are those who combine tactical importance with technical excellence. And for players, it underscores the importance of staying involved, those small passes and contributions add up, influencing not just the game but also how their role is perceived.
Now we will look into how transfer fees affect goals and goal-scoring ability. More specifically, we will focus on whether transfer fees serves as an incentive or a pressure builder when it comes to a player’s goal-scoring abilities.
Goal Scoring vs Transfer Fees
To understand how goal scoring ability is affected by transfer fees, we first need to understand what kind of factors would determine goal scoring. The first obvious one would be the player’s position. We thus control the player’s position in our models. Additionally, we also control for how the player position itself determines the impact of fee on goal scoring. For eg, a forward player might be more motivated or pressured to score goals with every additional 1$ of transfer fee he/she gets.
Next, we also understand that the outcome, goals scored itself, apart from factors like skill, player’s market value, match-time, their age etc, may also depend on pure luck. For eg, a certain player may be getting harder goal scoring opportunities compared to others, who might be in a position where they can score for eg, an easy open goal. While, controlling for this luck factor is hard, we have found a metric called xG (expected goals) which accounts for the probability that a goal scoring opportunity results in a goal. Thus, we will look at 3 different factors when understanding how, transfer fees affect goal scoring:
1. Expected Goals (xG):
Definition: A statistical measure that estimates the probability of a shot resulting in a goal, based on factors like shot location, angle, type of shot, and defensive pressure.
Purpose: xG quantifies the quality of scoring chances and predicts how many goals a team or player should score on average.
2. Goals Per Match:
Definition: The average number of goals scored by a player or team per game.
Purpose: A straightforward metric to assess scoring output, reflecting actual performance on the field.
3. xG - Goals Per Match:
Definition: The difference between expected goals (xG) and actual goals scored per match.
Purpose:
A positive value (xG > Goals Per Match) suggests underperformance (missed chances or poor finishing).
A negative value (xG < Goals Per Match) suggests overperformance (clinical finishing or exceptional skill/luck).
Expected Goals (xG)
We first look at expected goals to understand how the transfer fees of a player affect the quality of scoring chances created by them. First, we will look at a simple plot of the 2 variables to see if there is any visible relationship between them.
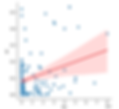
The scatter plot illustrates the relationship between two variables, with a positive trend indicated by the red regression line and its accompanying confidence interval (pink shaded area). Each blue dot represents an observation, and the clustering of points near the origin suggests that many observations have low values for both variables. The positive slope of the regression line indicates that as the x-axis variable increases, the y-axis variable tends to increase as well.
However, the spread of the points around the line shows considerable variability, suggesting that the relationship is not perfectly linear. The confidence interval widens as the x-axis values increase, reflecting greater uncertainty in predictions for higher values. This pattern highlights that while there is a general positive correlation, individual observations can deviate significantly from the expected trend, possibly due to unaccounted factors or randomness. The positive correlation is generally expected since, we should expect players with higher transfer fees to potentially work harder on the field, thus, creating better quality scoring chances.
To further tease out this relationship, we used the Boruta Random Forest Algorithm to determine what features could potentially act as good predictors of Expected Goals. The graph below shows different potential predictors of Expected Goals (xG) in order of importance:

The Boruta feature selection process identified key predictors for Expected Goals (xG), including start_ratio, xA (Expected Assists), matches played, penalty attempts, market value, and transfer fee. Thus, it is clear that players who start for more games, have higher assists, matches played etc are more likely more involved in the game and thus, create better quality chances. Additionally, both market value and transfer fee also turn out to be useful predictors. These features were confirmed as important contributors to the model, reflecting a player's consistency, creativity, and perceived quality. In contrast, variables such as age, minutes played, and disciplinary records like yellow and red cards were rejected as they showed little significance in predicting xG.
Firstly, we tried fitting a similar linear regression model to understand the impact of transfer fee on expected goals. Here, we already account for multicollinearity and heteroskedasticity. Additionally, we have controlled for several factors that may be influencing expected goals.

The model explains approximately 61.2% of the variation in xG, as indicated by the R-squared value, suggesting a strong explanatory power. Key variables, such as xA (Expected Assists) and Penalty_Attempted, are statistically significant (p < 0.05), highlighting their importance in predicting xG. Positional indicators, particularly Position[T.FW] and Position[T.FW,DF], also show significant positive coefficients, indicating that forwards and players in hybrid forward-defensive roles contribute more to xG compared to the reference category. Features like start_ratio and Mins approach significance (p < 0.1), suggesting that playing time and starting frequency may moderately impact xG.
Interestingly, variables like market_value, fee, and Age are not statistically significant, implying limited direct influence on xG after accounting for other factors. Disciplinary metrics like Yellow_Cards and Red_Cards also exhibit no meaningful relationship with xG.
However, this model does not capture cross relationships. For eg, one reason why fee may not be showing a significant impact on Expected Goals could be due to the presence of non-linearity and cross effects across positions. Thus, we conducted a RAMSEY RESET test and detected non-linearity in the model.
Now, we will run an improved model with interaction terms between Player position and transfer fee. There is also a strong reason to believe that transfer fee will not have the same impact across all player positions since forwards and midfielders may be more motivated to create goal scoring chances.

Adding the interaction terms, brings a more nuanced analysis. We can now see that while fee alone is not a significant factor, when combined with position, transfer fee has a statistically significant effect on Expected goals. More specifically, for Forwards, and players who transition between Forward and other positions, transfer fee has a statistically significant positive impact on Expected Goals. It is also interesting to note, that the impact of market value is no more statistically significant and so forwards may be motivated by short term financial gains to work harder and create good quality goal scoring chances.
Additionally, while the coefficient may seem insignificant and small, if we consider the marginal effect based on the values of transfer fees for forwards, here is the graph:
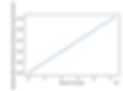
This shows that for the entire transfer fee span for transfers in 2020 Summer season, the marginal effect of fee on expected goals ranged from 0-0.25 which could be very significant. If we assume 38 matches for a player, the impact could be 0-9.5 goals per player for a EPL season which could be very significant. The average mean effect is around 0.07.
Thus, transfer fees for forwards seems to have a very significant impact on quality of goal scoring chances created.
Goals Per Match
We will now, look at the impact of transfer fees on the actuals goals per match. To do so, we will also control for the luck or the ease/difficulty of goal scoring chances using the xG metric.
As before, we first look at a graphical relationship between Goals Per match and transfer fee:

Based on the graph, we can clearly see a positive correlation between goals per match and fee. However, this may be potentially misleading since it doesn’t account for many factors including the positive correlation between xG and fee that we discovered in the previous section.
After repeating the procedure from the previous section, we discovered some interesting insights.

The positive correlation that we saw with the graph was mostly coming from Expected Goals. This can be clearly seen from the fact that xG appears to be strongly positively correlated with goals per match while the fee factor seems to be statistically significant. Another interesting insight that comes out is that there is some statistically significant effect of Fee on goals per match for players who transition between forward and defense, however, surprisingly this effect is negative. For pure forwards, too the effect while negative is not statistically significant at the 5% level.
This underscores an important point that transfer fee while it leads to players creating better quality chances, it does not make them deliver a higher output of goals. Additionally, the negative coefficient may reflect that with higher transfer fees, forwards might feel more pressured to score goals and thus, despite creating better quality chances, may lose composure due to the added pressure that comes with the higher fee.
We will further explore this hypothesis in the next section, by looking at the impact on the difference between xG - Goals Per Match and using it as a metric for finishing ability.
Finishing Ability (Expected Goals - Goals Per Match)
Next, we look at the impact of the transfer fee on the player’s finishing ability. We first, start by looking at the graphical relationship:

Here, we plot (Expected Goals - Goals Per match) vs transfer fee. While, there seems to be a concentration around the 0 fee mark, we can see a very weak to almost no correlation between fee and finishing ability. This could potentially be because the difference between finishing ability for different playing positions is not captured here or the effects of fee acting as an incentive or a pressure point, cancel each other out. Thus, now we will proceed to a formal regression same as earlier:

The regression analysis highlights the nuanced relationship between transfer fees and finishing ability, defined as the difference between expected and actual goals scored. While the overall coefficient for transfer fee is negative and statistically insignificant, suggesting that higher fees may sometimes correlate with lower finishing ability, interaction terms with playing positions reveal important context. For forwards, the interaction term (fee:Position) is positive and statistically significant, indicating that higher-fee forwards tend to exceed their xG more effectively. Similarly, higher-fee midfielders also demonstrate improved finishing ability, albeit with a smaller effect size compared to forwards. These results suggest that clubs generally invest wisely in players whose positional roles demand high finishing efficiency, though the broader negative relationship for fees could reflect overvaluation in cases where positional demands are less aligned with scoring outcomes. This underscores the importance of considering positional context when evaluating the impact of transfer fees on player performance.
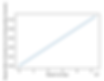
Additionally, when we look at the marginal effects for forwards, we can see that for the span of transfer fees, their finishing ability improved by 0-0.1, which could again, be significant. Thus, this shows that while fee may not have a significant or may have a negative impact on finishing ability overall, forwards often see it as an incentive and improve their finishing ability.
Key Insights
The analysis reveals a complex relationship between transfer fees and player performance metrics such as expected goals (xG), goals per match, and finishing ability (xG - goals per match). Transfer fees positively impact the quality of scoring chances created (xG), particularly for forwards and midfielders, as indicated by significant interaction effects. However, while forwards with higher fees tend to improve their finishing ability marginally, the overall impact of fees on finishing ability is negative and statistically insignificant, possibly reflecting added pressure or overvaluation. These findings underscore the importance of considering positional roles and psychological factors when evaluating the influence of transfer fees on goal-scoring performance.
Conclusion
The relationship between transfer fees and player performance in the English Premier League is complex and multifaceted. While transfer fees are positively correlated with certain metrics like passing involvement and the quality of goal-scoring chances (as measured by expected goals), they do not guarantee higher output in terms of actual goals scored or playing time.
For non-forward players, passing metrics emerge as a critical determinant of playing time, while market value acts more as an amplifier of other performance-related metrics than as a direct predictor. For forwards, higher transfer fees incentivize the creation of better quality chances but may paradoxically increase pressure, leading to diminished composure and fewer goals relative to expectations.
Ultimately, transfer fees reflect more than just a player’s technical abilities—they encapsulate factors like market demand, positional requirements, and reputational value. While they provide some insight into a player’s potential impact, they remain an imperfect predictor of actual on-pitch performance. This analysis underscores the importance of looking beyond financial figures and focusing on more nuanced metrics such as passing involvement, expected goals, and team compatibility to assess a player’s value and impact.
For fans, clubs, and analysts alike, this means appreciating the complexities of the modern game and recognizing that the true value of a player lies in a combination of skill, adaptability, and tactical contribution—not just the price tag attached to their transfer.