By: Andrew Weiner

Introduction
Lebron James dropped $36.8 million on his latest house in Beverly Hills in 2020, which included two guesthouses, a tennis court, and a movie theater. Fast forward to the NBA 2023-24 season, and the league’s highest earner is none other than Steph Curry, with a salary of over $50 million. It’s no secret that playing in the NBA is synonymous with fame and fortune, where the average player salary is just over $10 million this season. Franchises are willing to shell out hundreds of millions of dollars in player salaries every year to secure the assets they need for a good season. The most elite players receive huge contracts aimed at beating out competing offers.
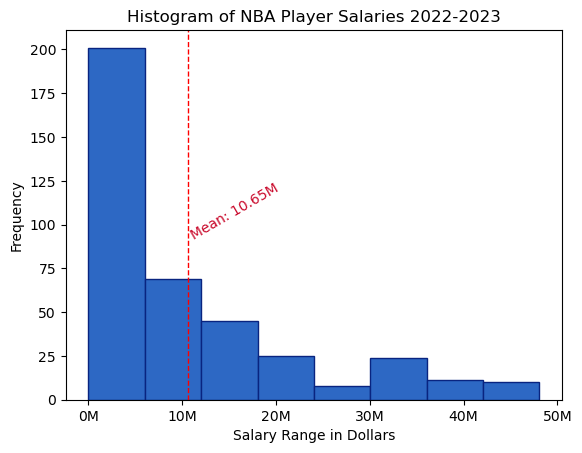
Although the mean salary is $10.65 million, the median salary is only $5.89 million. The most elite players in the league are few in relation to the league as whole, but get paid huge in comparison to their role player counterparts, which causes the data to be skewed to the right.
LeBackground
Signings in free agency have critical impacts on the fortunes of a franchise, whether it be good or bad. Golden State’s $54.3 million dollar contract acquisition of Kevin Durant in 2016 was a massive signing that forged a near unbeatable superteam, winning the NBA championship in 2016 and 2017 and solidifying a dynasty for several years. In today’s NBA, teams have found that having a star-studded roster with multiple high value-contract players is crucial for title contention. However, signing poor contracts that overpay players can set a franchise back, so teams need to invest their valuable cap-space wisely.
The NBA salary cap is complicated with many intricacies and exceptions that allow teams to exceed the cap in order to strategize with both retention of their current roster and acquisition of new talent. Namely, the Bird Rights exception enables teams to exceed their salary cap in order to re-sign their own free agents. Moreover, the salary cap has exploded in recent years from $109 million in the 2020-21 season to $136 million this 2023-24 season, putting more of the league’s income into the pockets of the players.
Salary cap exceptions and the increase of money in the player market has led franchises to pay top dollar for the most valuable and sought after players in the league. Despite a salary cap of $123 million in the 2022-2023 season, all teams aside from San Antonio exceeded that cap. This past season, the Clippers had a salary expenditure of $192 million, the highest out of the league’s 30 teams. They spent a sizable amount of caps-pace on superstars: Kawhi Leonard, Paul George, and Russell Westbrook, and they continued this trend this November with the acquisition of James Harden. But is splurging on an all-star lineup really worth it in terms of title contention?
Team Salary Analysis
It turns out money talks– to some extent. Last year, 14 of the top 17 teams with the most expensive rosters made it to the first round of the playoffs, along with Sacramento and Memphis being both first round exits with the 23rd and 26th most expensive rosters respectively. Generally speaking, the teams that paid more performed better, and with large differences in salary expenditures between teams, it looks like the less expensive rosters have a hard time faring against the more expensive ones.
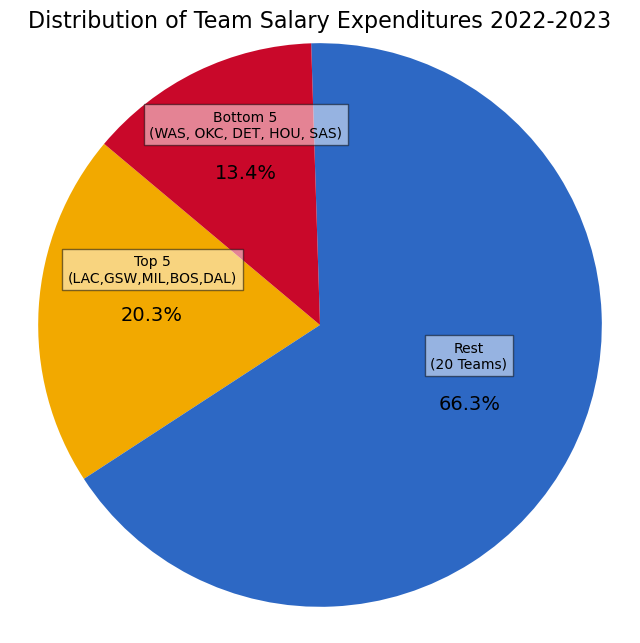
The LA Clippers’ roster cost is almost double that of the San Antonio Spurs’. High paying teams greatly contribute to the total salaries in the league at a shot for contention in the championship.
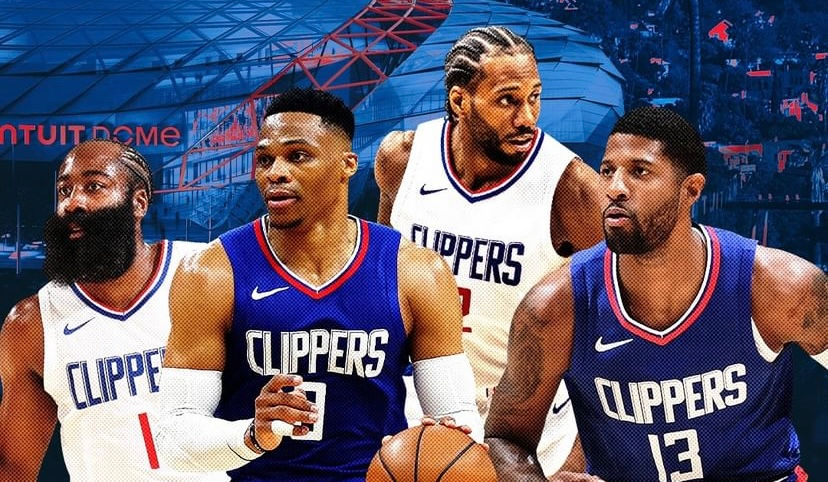
However, the Denver Nuggets– who held a commanding lead over the Western Conference last year and were crowned NBA champions– had the 8th most expensive roster. They were tailed by the aforementioned Memphis Grizzlies and Sacramento Kings last year who respectively finished second and third in the Western Conference with relatively cheap rosters. Moreover, the Miami Heat faced the Nuggets in the playoff finals with the 13th most expensive salary. A lot of teams outperformed what was to be expected of them based on salary– so where did some rosters miss out on value, and where did successful teams find theirs?
ML Linear Regression Model
A linear regression machine learning model was created to answer these questions about NBA salaries and player performance: what stats are most pertinent in determining a player’s salary? Which players were getting overpaid for their performance last year and which players deserved every penny and more?
Linear regression is a statistical method used to model the relationship between a dependent variable and one or more independent variables by fitting a linear equation to the observed data. In the context of predicting NBA player salaries, the linear regression model creates a function that takes certain stats from a player, and returns a prediction of that player’s salary based on the stats. The model creates this relation by being “trained” on the observed data, where it finds a best-fit equation for predicting player salaries based on stats by minimizing the mean squared error, or the average squared distance between its predicted salaries and players’ actual salaries.
Data Collection and Cleaning
Using BeautifulSoup’s HTML parser, player salary data for the 2022-23 season was scraped from HoopsHype, and both per game stats and advanced stats for NBA players were scraped from basketball-reference.
The first step was to clean the data for the purposes of the model. The data frames containing salaries, per game stats, and advanced stats were merged into one dataframe on the normalized character representation of NBA player names. Next, players who had no three point attempts had their three point percentage set to zero. Basketball-reference had multiple rows of stats for players who had been traded throughout the season, so rows with a player’s stats on different teams were omitted and rows representing the entire season were preserved. Then, players who had less than 20 games throughout the season were removed, as a larger sample size of games provides more meaningful insights into a player’s performance. Finally, players with two-way and 10-day contracts which pay substantially less than normal NBA contracts were removed. After cleaning, the dataset was left with 375 valid player entries.
Feature Selection
With the data cleaned, the next step was to find which player stats or features correlated the strongest with the salary of the player. The correlation matrix below contains player salary, stats, pergame stats, and advanced stats comprising 50 total variables. The top 16 features with the strongest correlation (absolute value) with player salary were retained, and all other values were removed. Below are the top 16 features and their corresponding correlation coefficients with the salary. Interestingly, the stats with the strongest positive correlation with salary were points per game (PPG), field goals (FG), and field goal attempts (FGA), indicating that either teams are willing to spend more on high scorers, or a high salary gives a player the opportunity to be an offensive centerpiece.
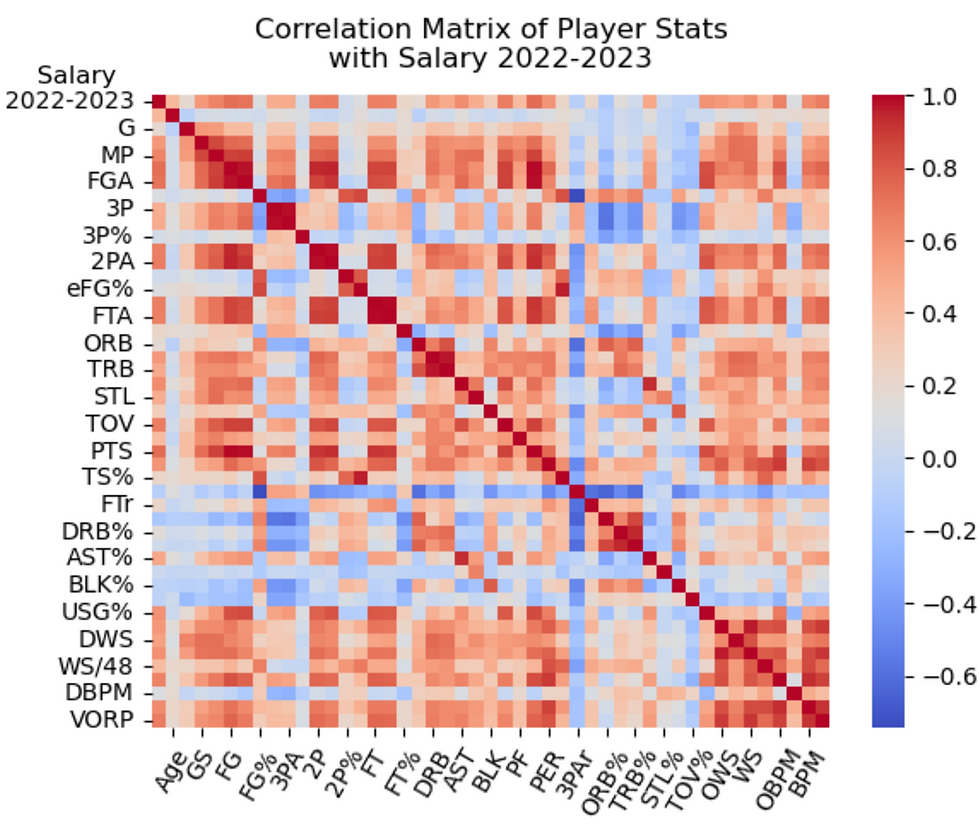
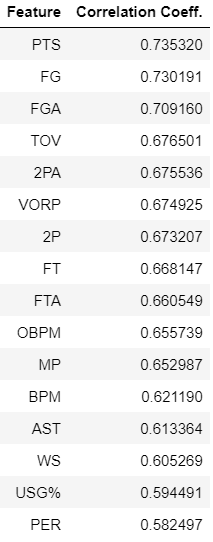
Next, the correlation of the 16 features themselves were analyzed. Advanced stats are often derivatives of per game stats, so some combinations of advanced stats and per game stats may be redundant to the model. The goal is to find a composition of features that don’t overlap in what they represent, but are each relevant to what the model is trying to predict.
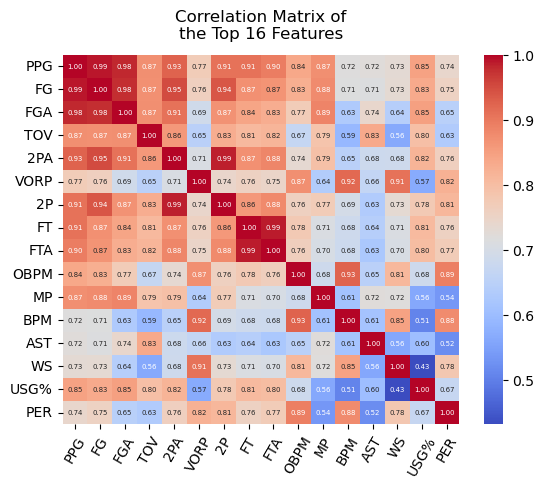
Features that expressed collinearity with other features were dropped. Most notably, PPG, FG, and FGA were all highly correlated, so PPG, the feature with the strongest correlation to the salary, was selected while FG and FGA were dropped.
After filtering for features with strong signal and low redundancy, the final features that the model will be trained on are: PPG, turnovers per game (TOV), assists per game (AST), value over replacement player (VORP), and player efficiency rating (PER). VORP is the estimate of the points per 100 team possessions that a player contributed above a replacement-level player, who is an average player that is readily available to replace them. PER is a standardized metric of overall efficiency of a player’s performance.
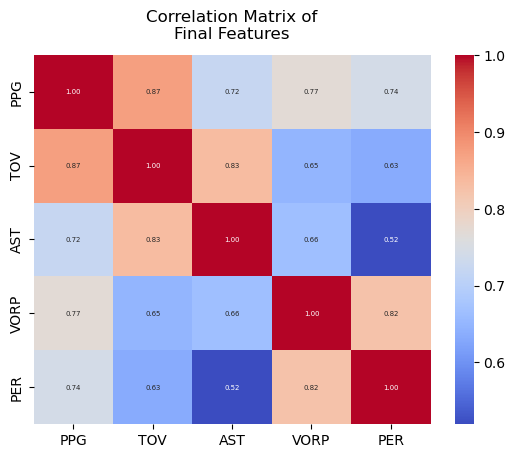
TOV was retained despite a relatively high correlation with PPG, because it had a high correlation with previous features that were not well correlated with any of the current ones.
Training the Model
Having filtered for the features, the data was then split into train and test sets by first shuffling the players and then taking %80 of the entries as the training set and the remaining for the test set. Next, the features were z-score normalized, to prevent features with larger scales from dominating the model. The features were then trained independently against the salary with ridge regression models of varying degrees. The goal was to find out how the different features relate to the target, and because the features were selected to avoid collinearity, they may exhibit different relationships with salary. It ended up that VORP and AST exhibited a slight quadratic relationship and the other features were linear, so a quadratic term for VORP and AST was added to the final model. The final model’s predictions had a mean absolute error of $4 million against the test set, meaning on average, the model’s predicted salary for a player was $4 million off the actual salary, which is not so bad given the magnitude of large NBA contracts and the model’s tendency to undervalue big name players and overvalue players with relatively small contracts in relation to actual salary.
Model Analysis
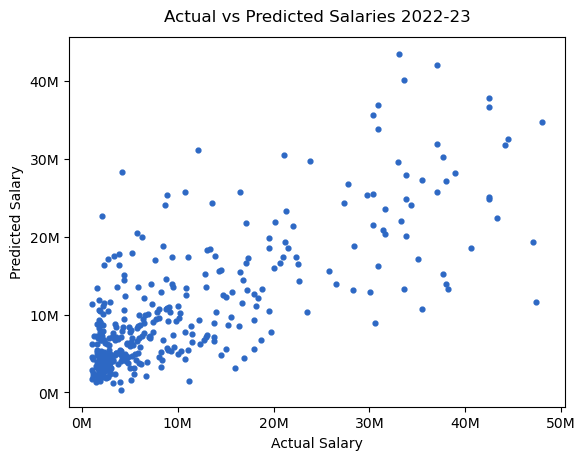
In the upper echelon of players, those whose actual salary is over $30M, the model lowballs on its predicted salary having only rewarded 3 players with a predicted salary over $40M, being Nikola Jokic, Luka Doncic, and Joel Embiid. On the other hand, it seems to think that players with lower actual salaries are typically more underpaid.
Is this indicative that NBA teams are overpaying their stars and neglecting their roleplayers? Not necessarily. The model was trained with salary data, so it really just predicts what a certain player should get paid based on trends in the NBA around how players get paid based on stats. General managers could see more value in players, especially superstars, because of their presence off the court, being big names that bring attention and income to a franchise, which was unaccounted for in the model. However, the model should represent how players should be getting priced based on key stats and past salaries, and it allows us to examine apparent mispricings in the player market.
Results
The Top 10 Players by Predicted Salary

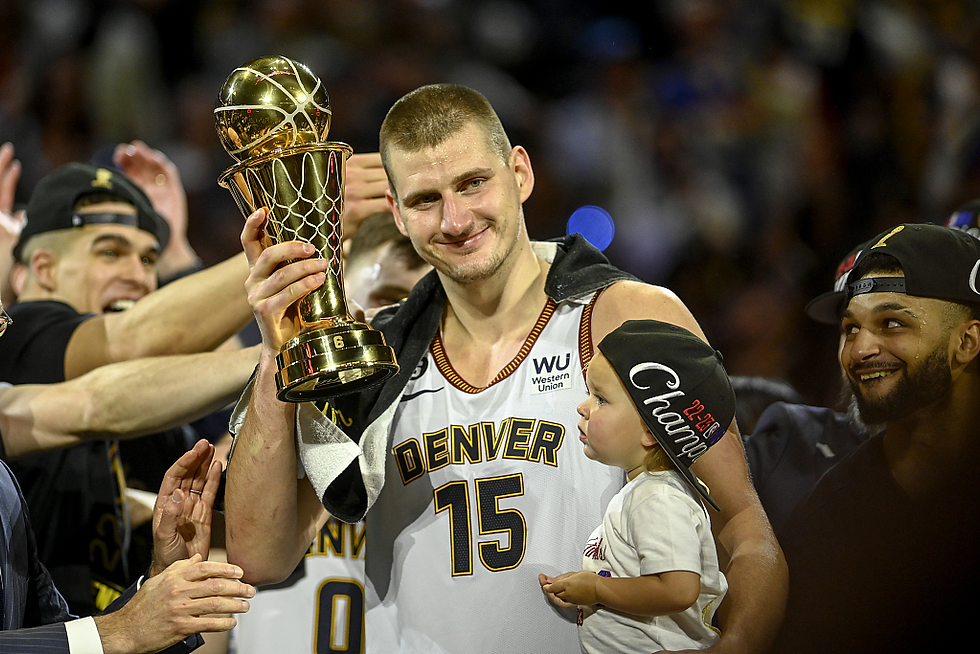
These are the players that the model deemed most valuable. To no surprise, all 10 players are the faces of franchises, the best of the best. It’s important to note that the model typically underpays big name players in relation to actual salary. Most notably, the two players that faced the highest negative difference in predicted salary vs actual salary were Lebron James and Steph Curry, who arguably have the greatest presence off the court in the NBA.
The 10 Most Underpaid Players by Salary Difference

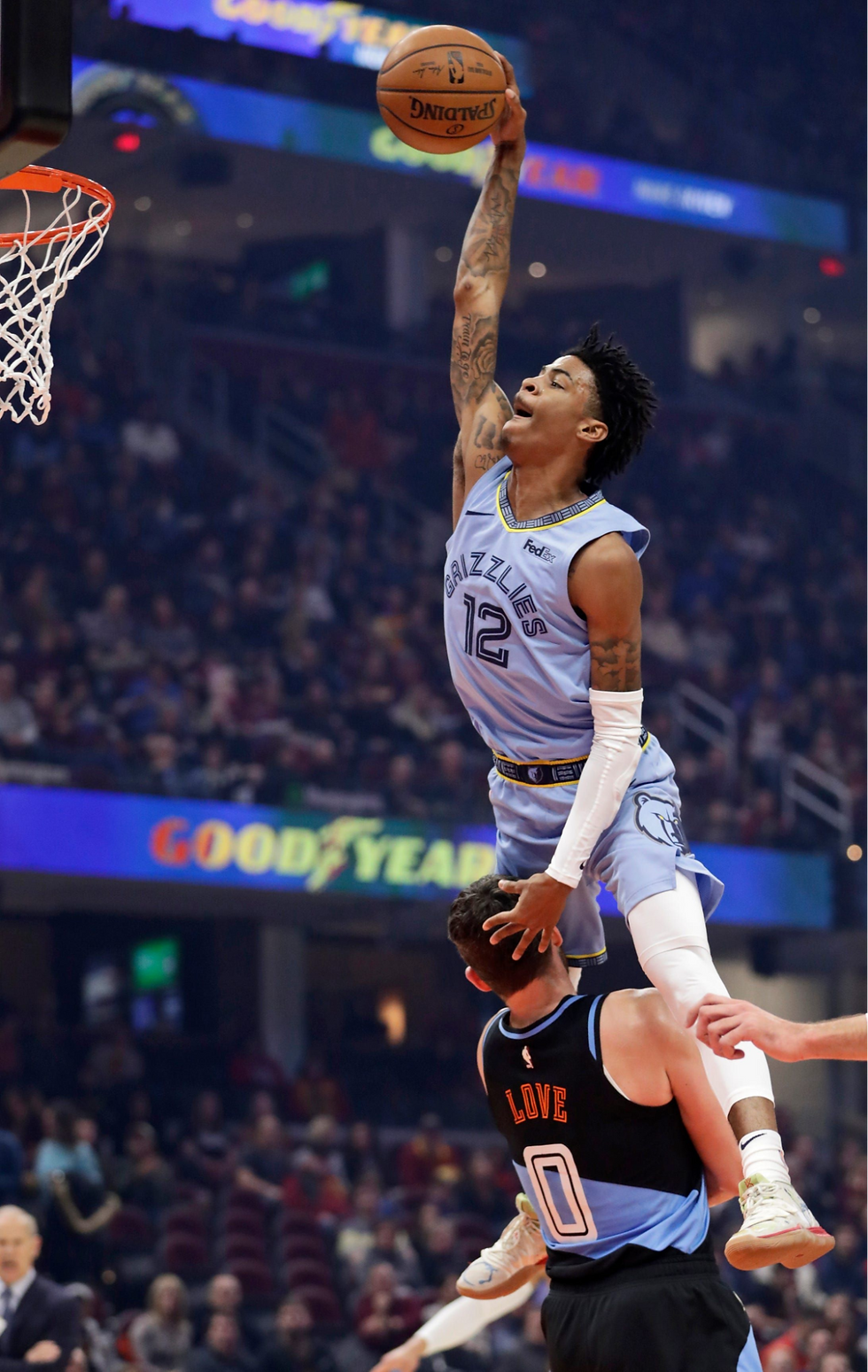
All 10 of these players are young, meaning they haven’t been able to get paid their full predicted value because of rookie contracts. This really shows who the best rookies are and the pay raises they can look forward to at the end of their rookie contracts. Tyler Herro, Darius Garland, and Ja Morant had rookie contracts that recently expired, and their predicted values were better reflected in their new contracts. For example, Ja Morant signed a 5 year extension contract with the Memphis Grizzlies, including $197,230,450 guaranteed, and an annual average salary of $39,446,090.
The 10 Most Overpaid Players by Salary Difference
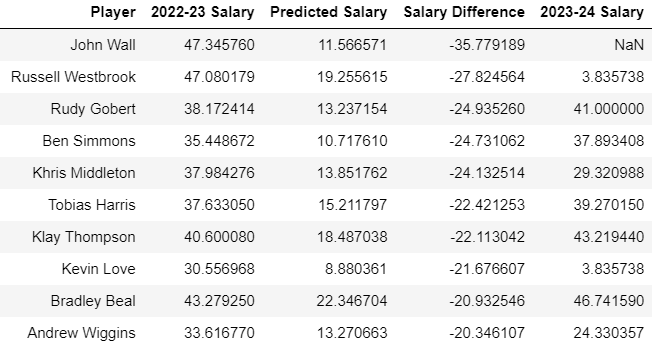
These are big names that
the model felt didn’t quite live
up to expectations in the
2022-2023 season. John Wall
received a $47 million
contract extension for the
Houston Rockets last season,
but didn’t make a return on investment due to issues with injuries and the Rockets’ lack of
focus on Wall, leading him to be bought out by the Clippers and eventually teamless this 2023-24 season. Russell Westbrook was facing large amounts of scrutiny during his time on the Los Angeles Lakers last season, because of a supposed lack of shooting ability. This led Westbrook to move to the Clippers where he took a massive salary cut and humbly assumed a more of a roleplayer position on the team. Most of the players on this list are maintaining high salaries, because they are in long-term contracts that extend into the current season.
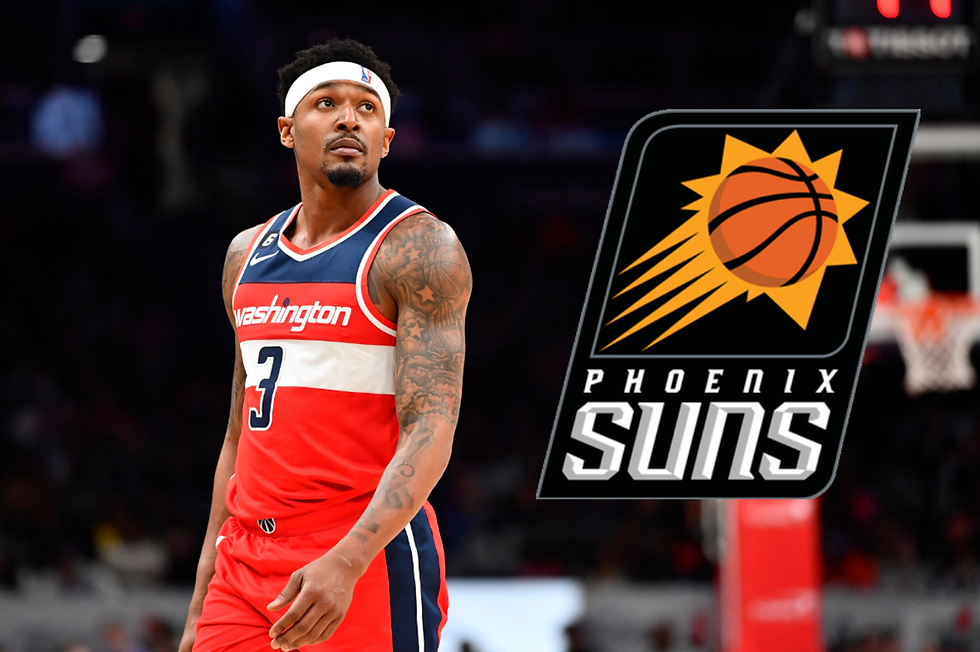
Bradley Beal, however, having averaged 23.2 points, 3.9 rebounds, and 5.4 assists on the Washington Wizards, didn’t play at a typical max-contract level. However, the Phoenix Suns traded for him and now have to pay the remaining $200 million on the last 4 years of his 5 year deal with an average salary of $50 million a year hoping to gain more value out of him than the Washington Wizards.
Sleeper Picks/Steals
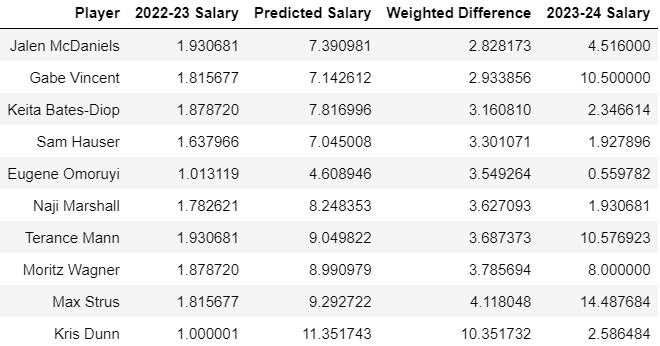
The “Weighted Difference” column was calculated by dividing the previous salary differences by a player’s actual salary. In effect, it is how many times more a player should be getting paid based on the model.
It was then filtered for players that are not on rookie contracts. All of these players, aside from Eugene Omoruyi of the Washington Wizards received a pay increase from the 2022-23 season into the 2023-24 season.
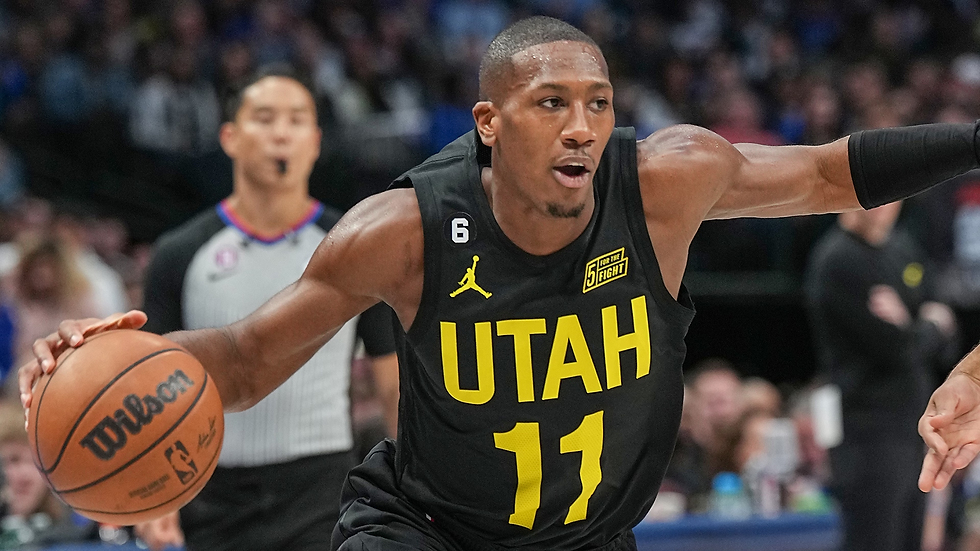
Kris Dunn of the Utah Jazz, the player with the highest weighted difference of 10.35, averaged 13.2 points, 4.5 rebounds, and 5.6 assists per game in the 2022-2023 season, numbers deserving of a raise. By the metrics of the model, the Utah Jazz found a steal, where Dunn actually has the highest predicted salary among the players in the table above.
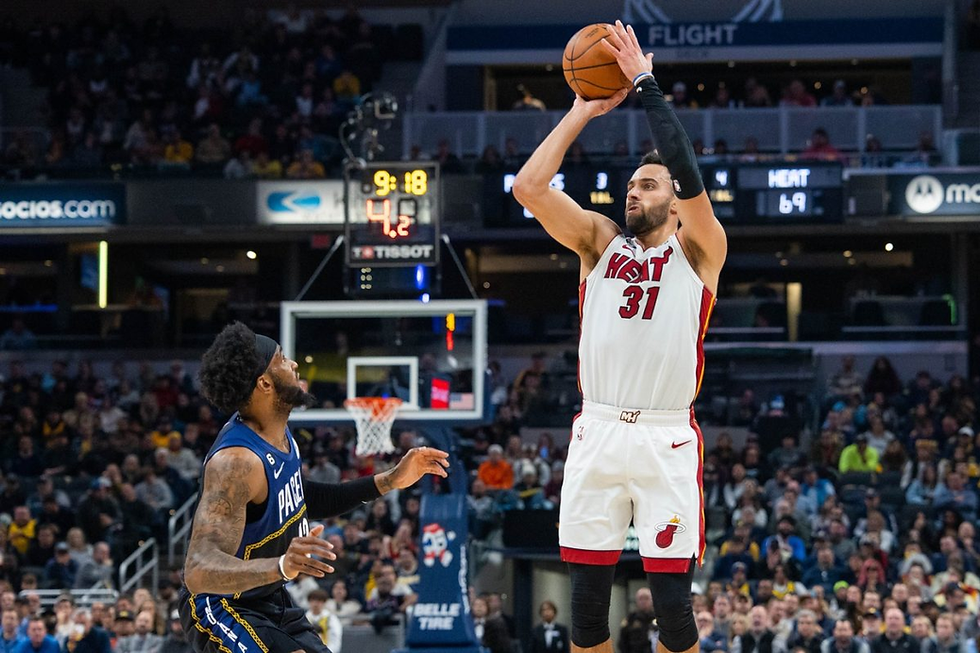
Max Strus, having been a key offensive player outside the 3 point line for the title contending Miami Heat, caught the eye of the Cleveland Cavaliers who offered Strus a 4 year $62 million contract, averaging a salary of $15.5 million a year, a huge upgrade from his previous $1.8 million upgrade from the Heat.
Similarly, Strus’ former teammate on the Heat last season, Gabe Vincent, signed a 3 year $33 million dollar contract with the Lakers. Like Strus, Vincent was recognized as an instrumental component of the Miami Heat’s performance that led to their finals appearance last season. This model agreed with this consensus, and reflected it by predicting a higher salary for these two players based on their performances.
Conclusion
Performing feature selection on a player’s season stats provided valuable insights into the factors influencing player compensation within the league. After training a linear regression model using the selected features and NBA salaries, the model was able to predict NBA salaries based on certain player stats throughout a season with reasonable accuracy.
However, the model would often overprice less valuable players and underprice more valuable players in relation to actual salary. An improved model would take into account postseason stats or how much market value off the court a player brings, and a classification machine learning model where players are grouped into salary ranges may represent the data better and more accurately depict the actual range of salaries in the league. In addition, there was a loss of information when stats with specific teams were omitted for players that were traded during the season. This is because being on different teams may have had effects on a player’s performance or salary that were unaccounted for.
Despite its limitations, when looking at specific deviations between actual salaries and the model’s predictions, it gave insight into how effective and valuable certain players are in relation to their income. Furthermore, many of the model’s deviations could be explained by looking at the broader context of a player’s current situation in the league. Moreover, many of the model’s predictions were reflected in changes in salary into the 2023-2024 season.
Comments