By: Arvind Kalyan
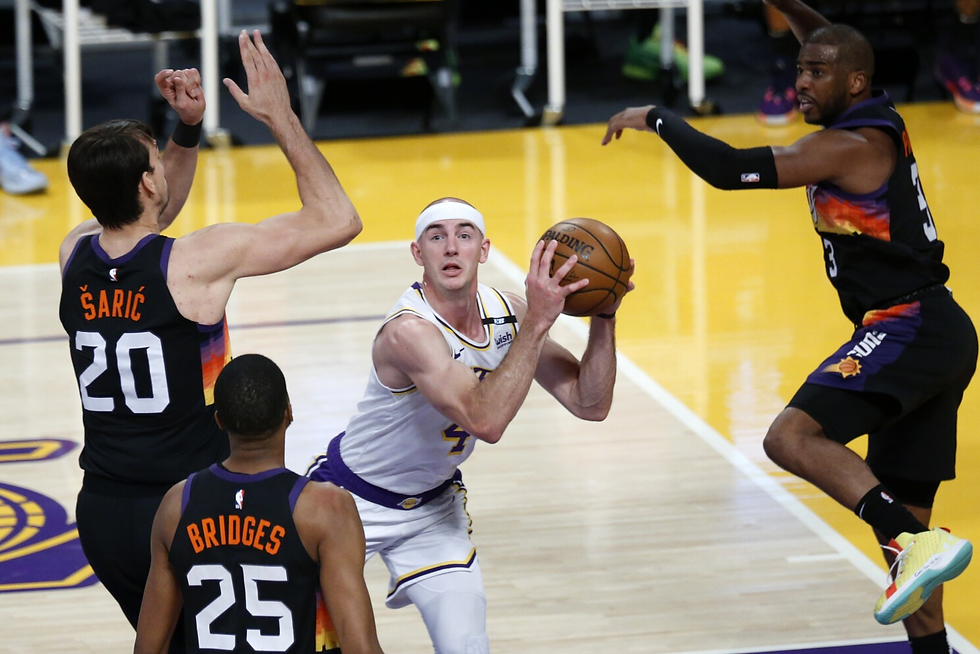
It’s no secret that the NBA has gone through its fair share of radical upheavals over the last few years. From the start of the small-ball Warriors dynasty to the revolution of the big man over these past two seasons, the game—and how front offices decide on building teams—continues to change.
In my previous article, I took a look at the most and least valuable skills for getting paid in today’s NBA. Now, following up on one of the future works mentioned, I’ll be conducting a similar analysis spanning the last six seasons of the NBA.
FEATURE SELECTION:
I found myself satisfied with how the stats chosen in the first article matched up with specific skills when it comes to filling out an NBA team. New for this study, however, is the addition of age. Age isn’t a skill, of course, but since contract scales are different for players in different service-time tiers, I chose to include age as a feature to prevent it from being a confounding variable in the analysis.
I gathered these stats for all players from 2016 onwards, compiling six separate tables filled with data. Then, using historical contract data from HoopsHype, I matched each player’s stats with their salary for that season. Unfortunately, since BasketballReference doesn’t aggregate its salaries historically, and Spotrac’s (useful) data lives behind a paywall, I lost the ability to track how each player was signed.
Here are the features, again listed with an official definition from NBA.com if necessary.
OFFENSIVE
Scoring:
Less than 5ft FGM
10-14 ft FGM
15-19 ft FGM
20-24 ft FGM
3PM
Screen Assists: The number of times an offensive player or team sets a screen for a teammate that directly leads to a made field goal by that teammate
Rebounding:
Offensive Rebounding
Defensive Rebounding
Rebounding
Passing: ASTADJ (Adjusted Assists): The total sum of a player or team's assists, free throw assists, and secondary assists
Passes Made
DEFENSIVE
Rim Protection:
Contested 2pt shots
Blocks
Perimeter Defense:
Contested 3pt shots
Steals
Hustle:
Deflections
Loose Balls Recovered
ATHLETICISM:
Average Speed
Average Speed (OFF)
Average Speed (DEF)
OTHER:
Age
DATA PROCESSING:
As before, the first step is normalizing each stat across minutes played, accounting for the “sunk-cost” bias that arises from playing higher paid players more minutes, even if their actual skills don’t necessarily call for it (see: Russell Westbrook, 2022).
Then, in order to counter issues with small samples, I removed all players who played less than 350 total minutes across each year. I’ve found this 350-minute threshold to be quite useful, as it cleaved the player pools from each season to between 380 and 390 players, with leaderboards that seemed a bit more reasonable.
Finally, I used the same logarithmic scaler on the data, bringing the distribution to a slightly more normal shape.
Using the above stats as features, I used each player’s salary as the target variable for the model. With each of the salaries, however, a bit more processing was required.
I attempted to balance out the super-max contract, which can only be signed by the small subsection of players who have earned significant accolades, accrued at least eight years of service time, and re-up with their original team. I clipped player salaries across the six seasons to an upper bound of $40M, placing players like Russell Westbrook and Steph Curry, who are each set to earn upward of $45M as a result of contracts with their original teams, and Paul George and Kevin Durant, whose salaries hover around $40M due to their decisions to sign elsewhere in free agency.
Finally, in order to exclude players playing on their rookie contracts, I applied a filter to remove players under 24 years of age who made less than $12M each year. Admittedly, this is a bit broader of a stroke than I would have preferred since it essentially omits any extremely young players who happened to actually sign a 10M contract after their rookie deal expired. However, as mentioned before, I wasn’t able to access each player’s means of signing (rookie contract, mid-level exception, etc...). Thus, under the assumption that these players are far and few between, I decided upon this filter as the most efficient way to cut away players like Luka Doncic or Jayson Tatum, whose immense value could not possibly be represented by the sub-$10M AAV contracts the two played on prior to signing max-level extensions.
MODEL:
I re-upped with the same XGBoost Classifier before, using the normalized stats listed above as the features and the scaled salary as the target variable.
Essentially, the XGBoost Classifier trains multiple decision tree models sequentially, using the error residuals of the previous models’ results to influence future models, then aggregating these models together in the end. Once the final model is obtained, we can calculate an F-score for each attribute, denoting its number of appearances in the model and therefore, representing its relative feature importance.
The most important skills, represented by these features, are the ones that are most “important” toward the salary that each player makes, or the target variable used in classification. Note that the importance of each of these features are listed in order of magnitude, so their actual association may be either positive or negative.
FINDINGS:
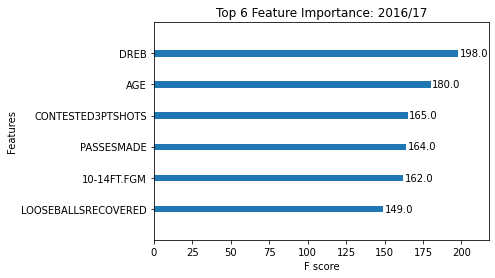

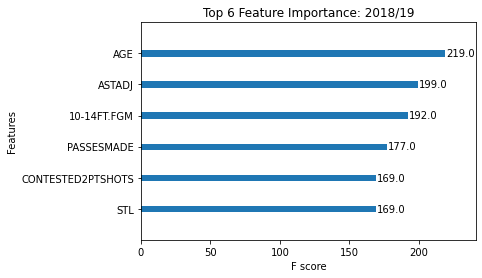
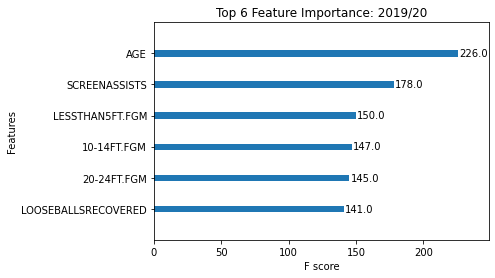
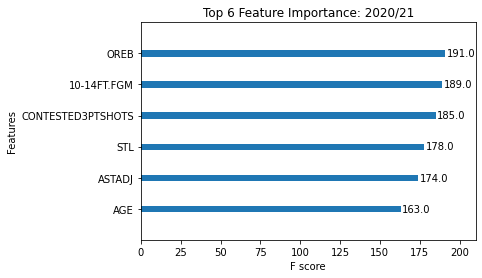
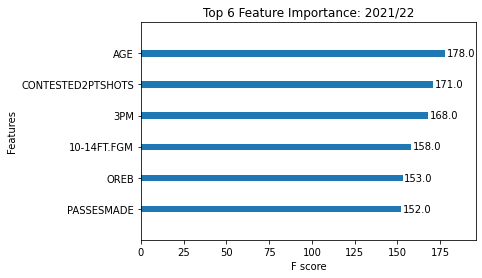
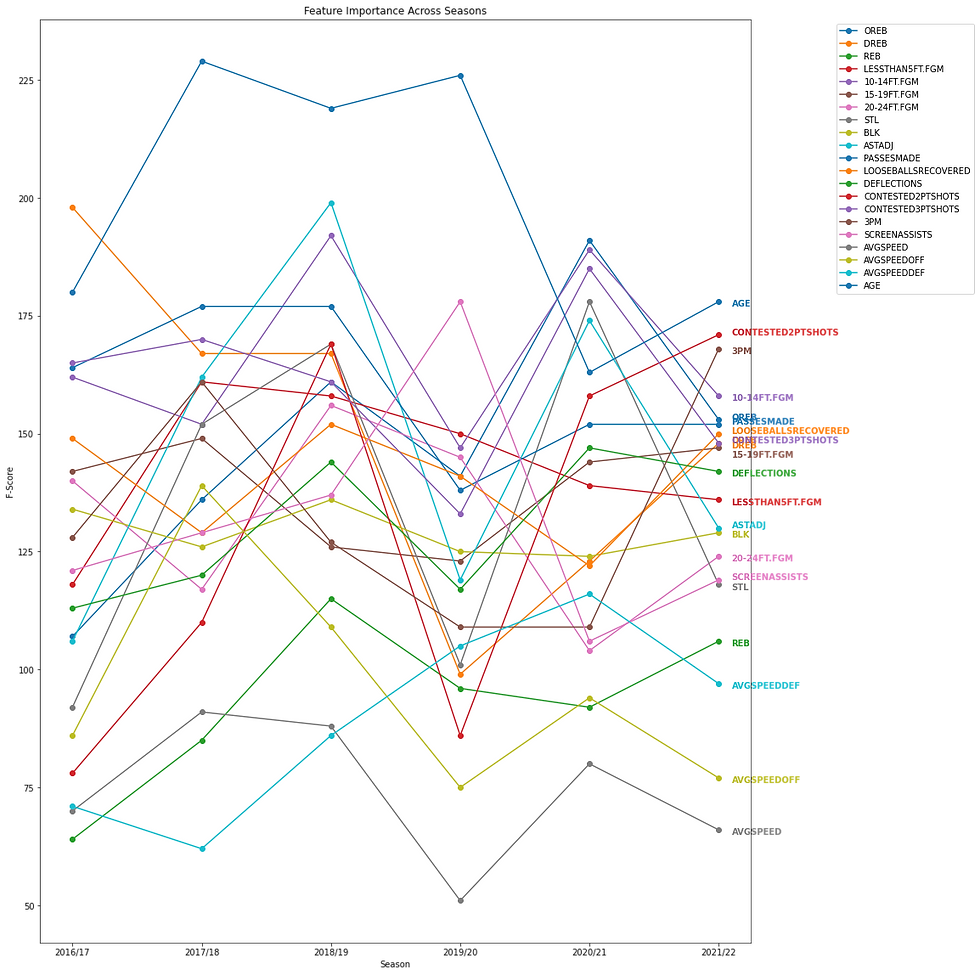
The most notable characteristic of the data is the consistent importance of age across the years. With how basic contracts scale on service time (essentially, age), it stands to reason that age would have a strong association with getting paid. There’s also a bit of a self-selecting effect here, as the only players who stick around at older ages are also the ones good enough to be paid more. I decided to extract it as a feature and display the other top five features instead.
3PM
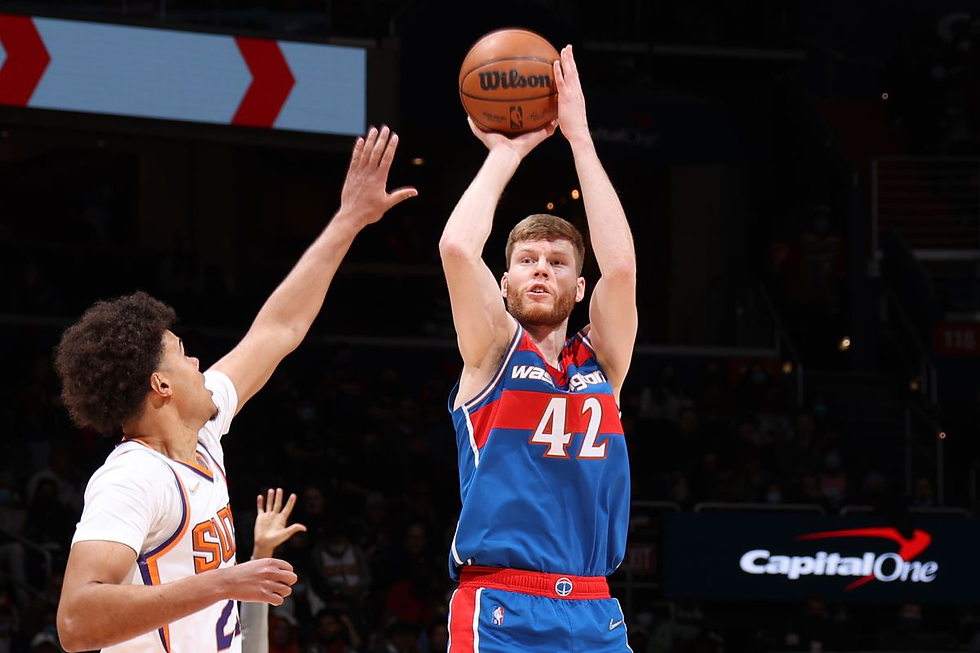
In looking at the 2021/22 season, the sharp rise in importance placed on 3-point shooting leaps off the graph. The NBA sits squarely in its golden age of 3-point shooting and floor spacing, evident in the rising rate of attempts from beyond the arc over the last few years.
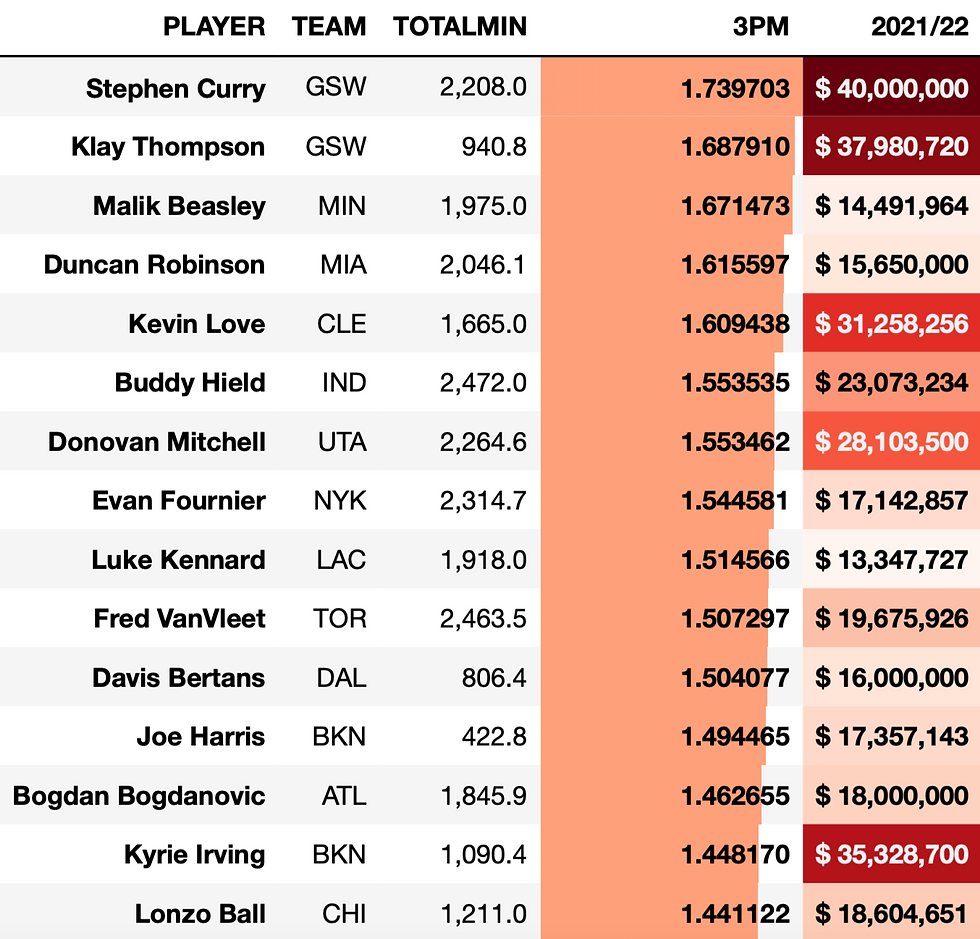
Aside from Stephen Curry and Klay Thompson—the leaders of the 3-point revolution who have been paid accordingly—teams have been able to acquire shooters without breaking the bank. Malik Beasley, who finished third in adjusted-3PM, made just under $15M per year, while the rest of the leaderboard is littered with players with salaries in the $15M-$20M range. We can owe this trend to the influx of 3-point specialists, and the general league focus on developing a 3-point shot. The market has adjusted to the saturation, and today, teams have been able to get elite shooting from distance on a budget. The skill simply isn’t as special as it once was.
DREB
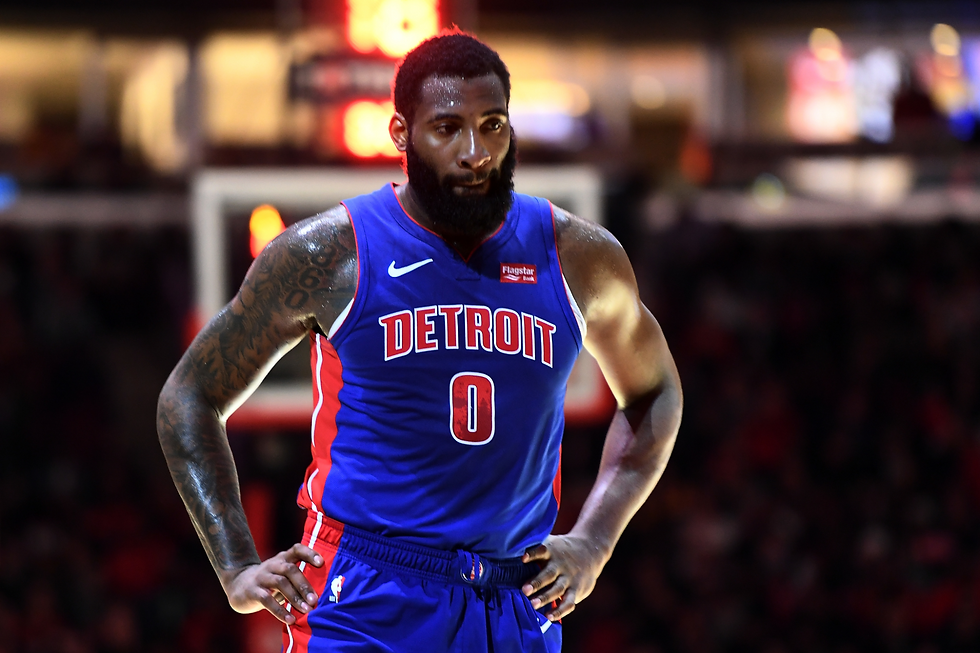
One feature that has remained relatively consistently high in importance is defensive rebounding. In 2016, after the historic cap-spike, teams (for whatever reason) began to shell out large contracts for good defensive rebounders. This may be a product of the specific players that happened to hit the market during the historic 2016 free agent frenzy—Bismack Biyombo, Joakim Noah, Dwight Howard, Andre Drummond, and Hassan Whiteside, all members of this class, make the list.

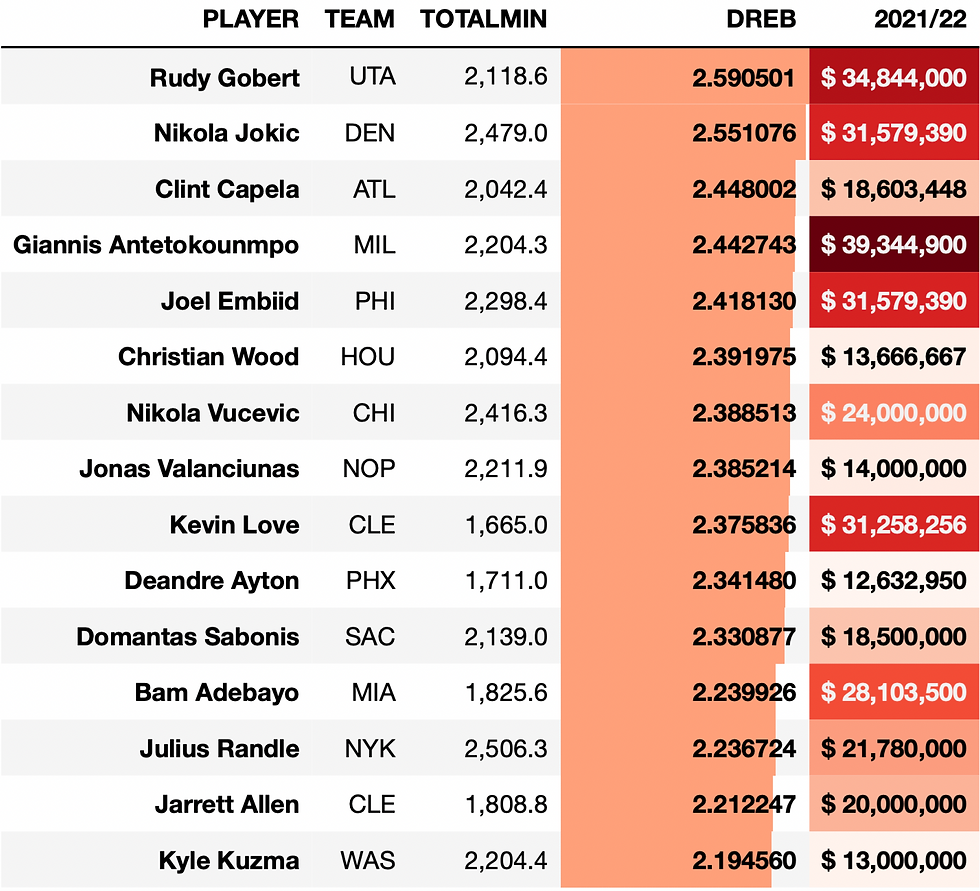
Fast forward to 2021, however, and it appears teams have been able to find defensive rebounding at relative bargains. The true elite bigs like Rudy Gobert and Nikola Jokic are paid handsomely, of course, but players like Christian Wood, Jonas Valanciunas, and Kyle Kuzma have provided strong rebound production while making under $15M. A look at the heights of the players listed in either chart reveals an interesting sub-trend. For the most part, the players atop the 2016-17 leaderboard are tall, rim-running centers. The 2021-22 list, however, features more undersized stretch-bigs who, while still providing strong defensive rebounding abilities, operate in different roles offensively than their counterparts from 5 years prior. All this speaks to the devaluation of the 7-foot, plodding center—the Andre Drummond archetype, if you will—as the game has shifted to the 3-point line and small-ball lineups.
Conclusion:
As mentioned above, however, one of the confounding factors with all of this is the 2016 cap spike, which saw many players receive contracts that.... did not befit their true talent, to be diplomatic. This then had a ripple effect, where the crop of players paid as part of that free agent class skewed data through the remainder of their contract. Ultimately, however, this study reveals the profound effect that the 3-point revolution has had on the modern NBA market. The league is saturated with players who are, at the very least, adequate from beyond the arc, so the value of a true marksman from 3 is dampened. Similarly, we see the league’s shift from true, big centers to cheap, undersized centers in the defensive rebounding section—another side effect of the recent trends in the NBA. It’s a fool’s errand to predict just where the game will be another five years from now as it continues to evolve and grow, but until further notice, the 3-point revolution is here to stay. Perhaps a shifting of the arc a few feet back, or the addition of a 4-point line, will be the first true impetus against the movement.
Комментарии