By: Jun Yu Chen and Eric Xia

Introduction
We all know that injury is an inevitable, yet unforeseeable part of basketball. In particular, in a professional league that is as competitive and physical as the NBA, we have witnessed career-ending injuries that ruin the bright future of many rising elites. On the other hand, we also see superstars who overcome severe injuries with unbelievable comebacks. One question naturally arises: what factors determine players’ injury comeback performances? The obvious observation is that injury comebacks depend on many explicit and implicit features such as Age, Injury type, Rehabilitation, Height, and even psychological factors like confidence level. Such complexity and interactions of different factors make it manually impossible to derive or hypothesize a straightforward relationship about injury comeback performances. But in real life, as passionate NBA fans, we always wonder how well popular NBA players come back from injuries.
In this article, with the assistance of machine learning models, we strive to find a way to unveil such a multi-level relationship and make a prediction about injured NBA players’ return performances in the 2022-2023 NBA season. In our case, we chose to predict Zion’s comeback performance in terms of Efficiency Rating (EFF) with some case study analysis. The choice of subject is due to personal preference and the fact that Zion is a rising star who is returning from an out-of-season injury.
Why EFF?
Among all the box score statistics, we chose Efficiency Rating (EFF) as our standardized metric because it provides a well-rounded and comprehensive mechanism for evaluating players’ performance. EFF considers different elements of the game and is calculated by adding up all of the production stats (points, rebounds, assists, steals, and blocks), subtracting all missed shots (field goals and free throws) and turnovers, then dividing by games played.
Data Source:
In order to examine the effect of different factors on the efficiency score of injured players, we need a list of injured players and the type of injuries they had, the demographic data of the injured players such as age, height, weight, and the efficiency of these players before and after their injury.
To acquire these information, we found three separate datasets and integrated them for our analysis. The first dataset “NBA Injuries from 2010-2020”, posted by Randall Hopkins on Kaggle, includes detail on every injury in the NBA from the beginning of the 2010-2011 season through the end of the 2019-2020 season. The second dataset “NBA Players”, posted by Justinas Cirtautas on Kaggle, contains two decades of demographic and biographical data on all NBA players. The third dataset we used was taken from the website “Basketball Reference” that contains the game statistics of all players from the 06-07 season to the 21-22 season.
Variables:
Year: Year of the season. Since the NBA season stretches over two calendar years, the year given is the first calendar year for that season. For example, the year for the 2010-2011 season would be 2010.
Player: the name of the players, in the order of first name and last name.
Pos: The role that the player plays on the court. C is center, F is forward, G is guard, SG is shooting guard, SF is small forward, PF is power forward, PG is point guard. When there are two positions listed, the one in the former is the position that the player normally plays
Team: The NBA team that the player plays for.
Injury Note: Details about the injury that the Player had. As a reminder, in our data analysis, we only filtered season-ending types of injuries since we want to limit our study to players who come back from significant injuries.
Height: The height of the player in cm
Weight: The weight of the player in kg.
Age: The age of the player in years.
Prev_EFF: The first available efficiency rating(EFF) of the player before the injury.
After_EFF: The first available efficiency rating(EFF) of the player after the injury.
EFF_Diff: The change in efficiency rating(EFF) of the player. A positive value indicates an improvement in efficiency rating, while a negative value indicates the opposite.
Data Cleaning:
Injury list: We started with a raw injury dataset that lists the injury notes and matched it with the corresponding NBA players, their demographic, and game data on two larger NBA datasets.
From the second dataset, we obtained the height, weight, and age at the time of injury of the injured players.
From the third dataset, we calculated the Efficiency Rating of each injured player using their game stats and determined the first available Efficiency Rating both before and after their injury. We then integrated this information into the first dataset and dropped the players with any missing data.
Here are the top five rows of our cleaned injury dataset.

Data Visualizations:
We also created several plots to visually explore how different factors, including Age, Position,
Weight, and Injury Types, impact the change in Efficiency rating.
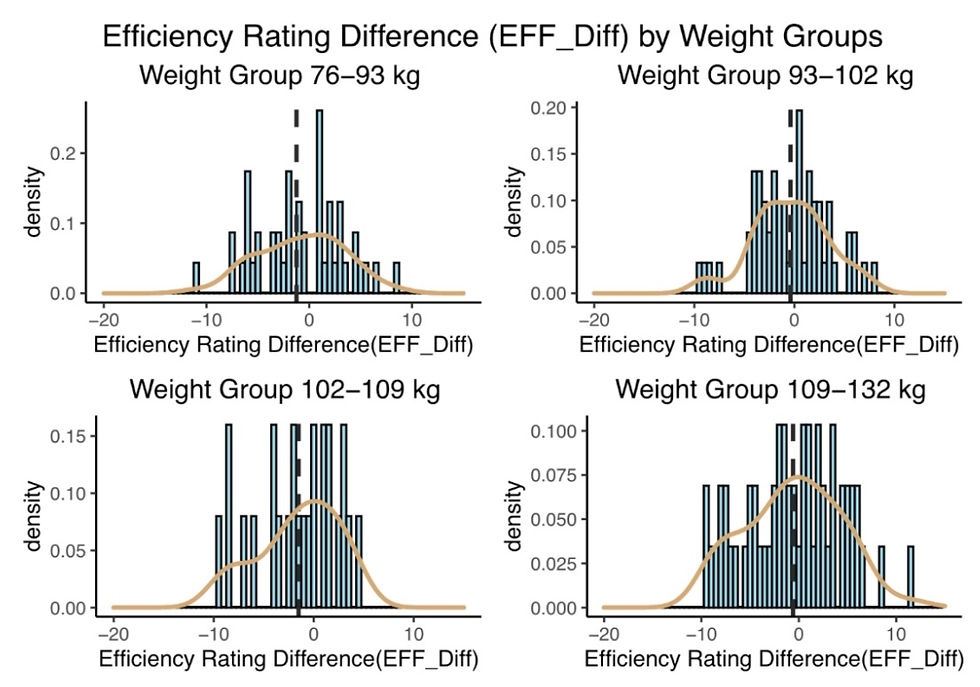
According to this graph, no obvious patterns can be identified, as for all Weight groups the change in Efficiency Ratings tends to be slightly negative. The change in Efficiency for Weight Group 102-109 kg, which is the 50th to 75 quantiles, tends to be relatively more negative.
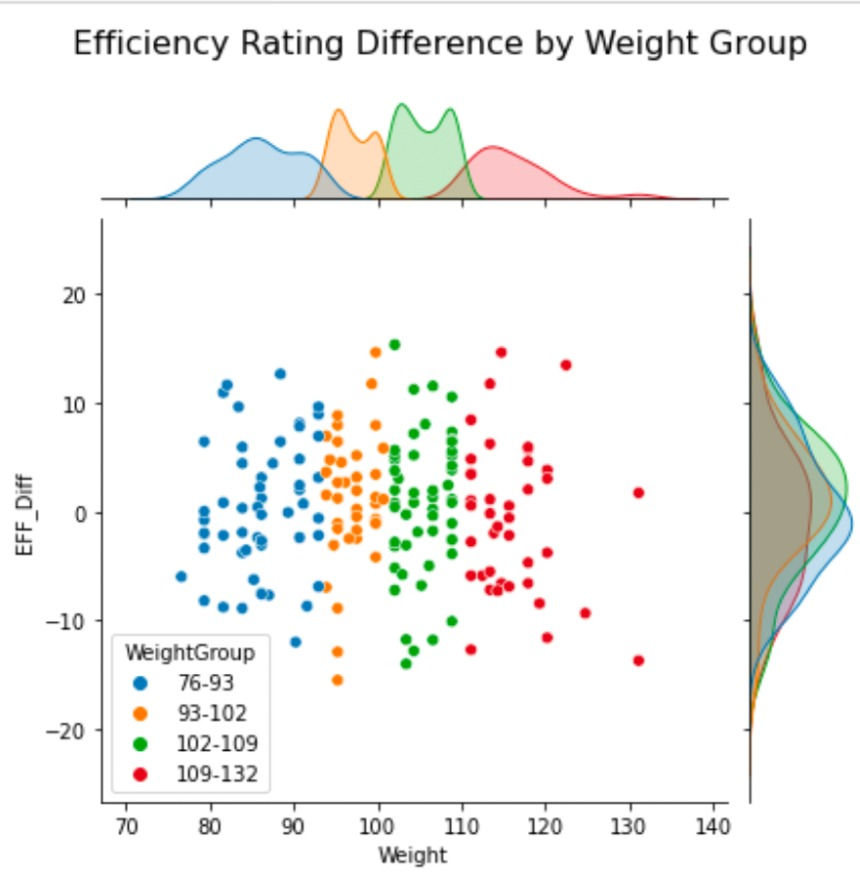
Graphing this relationship with a scatter plot confirms our previous interpretation as the dots split by weight groups tend to be unorganized and scattered around, indicating no obvious relationship.
Interpretation:
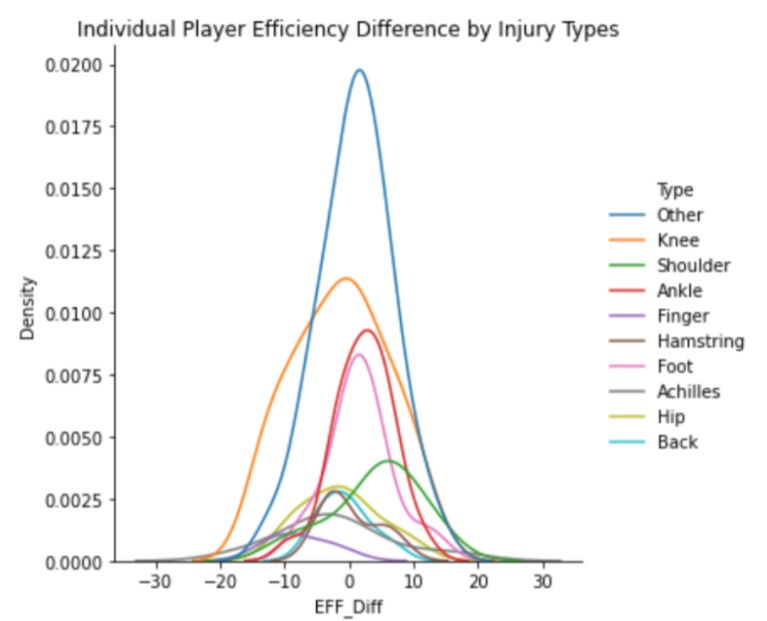
In this graph categorized by injury types, we observe that players with shoulder, Foot, Ankle, and other injuries tend to have more positive comebacks, while players with, Hamstring, Finger, Knee, Achillie, Back, and Hip injuries tend to have worse performances, with Finger and Achilles injuries being the worse.
Most of these results align with our expectations, as injuries on the negative spectrum, such as the Knee and back, tend to be more recurrent and usually deal with excruciating pain. Foot and ankle Injuries are musculoskeletal and tend to heal more completely with appropriate therapies and recovery training. Achilles injuries tend to have a more severe impact on comeback performance as structural changes that occur in the tendon due to degeneration are largely irreversible. Also, out-of-season injuries dealing with Achilles are more likely to tear than swelling or lighter symptoms.
The fact that finger injury has the most negative EFF difference in this graph is counterintuitive as finger injuries are usually minor. However, season-ending finger injuries are perhaps on the other spectrum, possibly with more severe symptoms such as deformations and bone fractures. Moreover, severe finger injury may alter how comfortably players shoot the basketball, possibly leading to changes in shooting form and confidence level that have both negative psychological and physical impacts in the short term. Since we only record players' first available EFF after injury, long-term impact requires additional exploration.
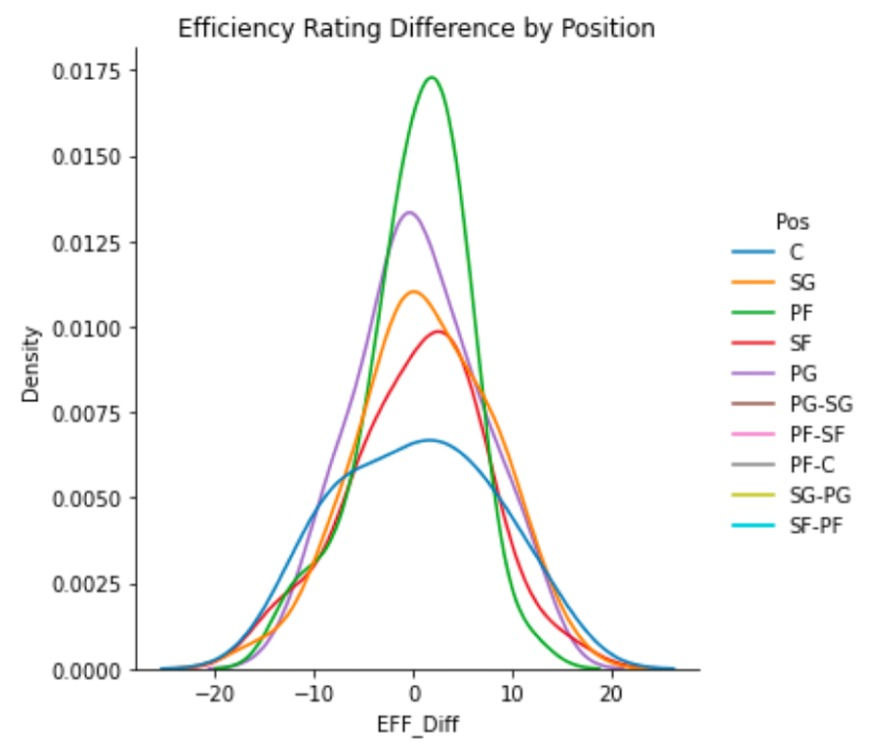
When we categorize by position, we observe that power forward, and small forward tend to have more positive EFF differences, indicating better comeback performance. However, point guard and shooting guard tend to have slightly more negative EFF differences, indicating worse comeback performance. There is insufficient evidence to explain such minor differences, but one explanation is that forwards tend to have better physiques and more muscles that help them to minimize tissue damage and irreversible impact from injuries. Moreover, such differences in EFF can be a product of the post-injury roles player play, as players of different positions change their playing style differently after injuries.
As a general pattern, wings tend to play more off-ball and and less intensive roles post-injury while point guards are on ball duty almost all the time, so they still have to rely on their athleticism to run the rim and facilitate team plays. As an example, Blake Griffin transitions from dunking on everyone to shooting more and handling the ball more in transition. As another example, Kevin Durant also changed his game style from directly attacking the rim to more post-ups and relying more on his footwork.
He came in dunking on everyone and being really close to the rim. Now he shoots a lot more where that's his focus over big dunks and rim attacks. He also handles the ball in transition quite a bit as one of those hybrid forwards.
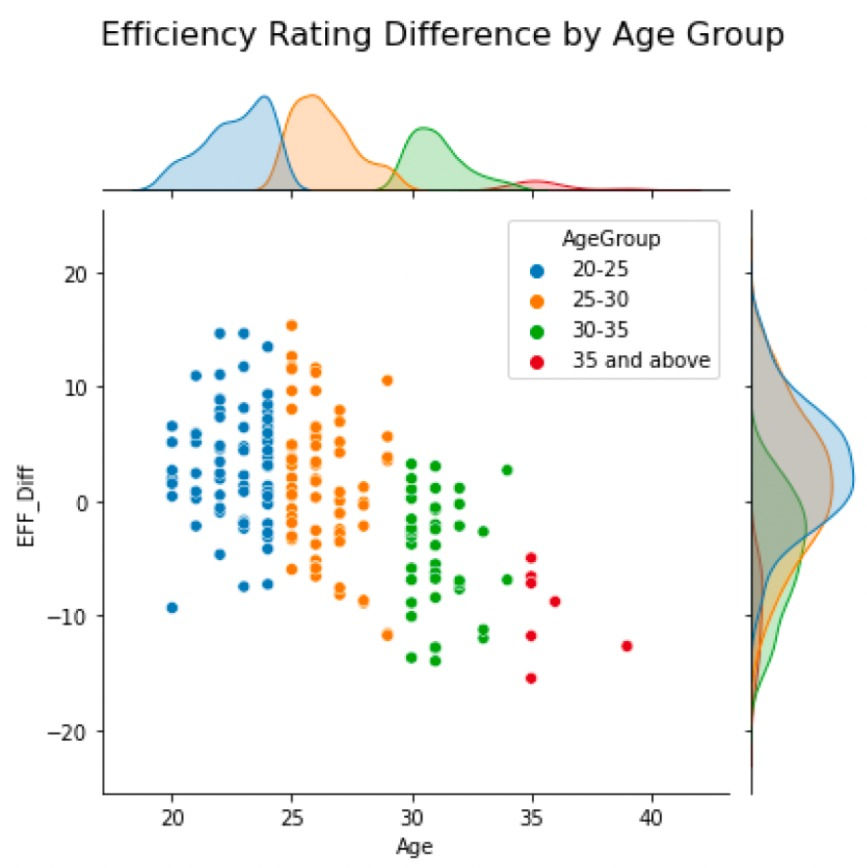
When we compare Age groups with EFF differences, the relationship is quite linear. Clearly, EFF difference is negatively associated with age. As age increases, EFF difference decreases correspondingly. This also aligns with our expectations. From a medical and physiological perspective, older athletes tend to experience loss of flexibility, muscle strength, and functional decreases in energy replenishment, which makes it more challenging for them to recover fully from severe injuries.
Machine Learning Model And Results:
Regression model:
The first model we tried to build is a regression model as we wanted to predict the Efficiency Rating after the time of injury using various features including the player’s height, weight, age, previous EFF, and position. Our ultimate goal is to have a machine learning model where if we feed Zion Williamson’s information into the model, we will be able to predict how well he will do in the current 22-23 season in terms of his Efficiency Rating. Before we built the model, we further cleaned and prepared our data in order to improve the performance of our model. In particular, we first hot encoded all the categorical data including injury type and player positions into 1s and 0s. We then normalized the data into the same scale between -1 and 1. Finally, we split the data into training and testing sets with the after EFF variable being the value that we are trying to predict. Now that we have prepared our data, we are ready to build the model.

Here is a screenshot of the top 5 rows of our data after data cleaning preparation.
For our model, we constructed a three-layer sequential neural network model using TensorFlow, an open-source software library for machine learning and artificial intelligence. After training the model on our training dataset, we fitted the model onto the testing set to see how well our model can predict a player’s Efficiency Rating after their injury. To evaluate our model’s performance, we used mean absolute error as the metric. Mean absolute error measures the mean of the absolute values of the individual prediction errors over all instances in the test set. In this case, the resulting MAE tells us how much on average our model’s predicted EFF after a player’s injury differs from his actual EFF after the injury. After several iterations and parameter tuning, our neural network model resulted in a mean absolute error of 5.2135.
To contextualize our finding, we’ve plotted the training/validation losses as well as predictions vs. true value.
We see a rapid decrease in both training and validation losses as our model begins to train on the training dataset. But the validation loss reaches a standstill after around 17 epochs, which suggests that any more training would only lead to overfitting. Additionally, as we can see from the predictions vs. true value of EFF graph, our model does not seem to perform that well since it tends to both underestimate and overestimate a lot of the data. The resulting correlation coefficient (R^2) from the predictions vs. true eff relationship was 0.05022.
Seeing that the neural network model did not give us very good results, we decided to take on a different approach and used the XGBoost regression model for the predictions. XGBoost is a machine learning library that implements optimized distributed gradient boosting machine learning algorithms under the Gradient Boosting framework. With this powerful tool in our hands, we fitted the XGBoost regression model to the same dataset and this time, we were able to get a MAE of 3.9456. Moreover, the r^2 between predictions and true EFF rose to 0.4957, making us more confident that this model would perform better at predicting Zion Williamson’s EFF in the upcoming season.
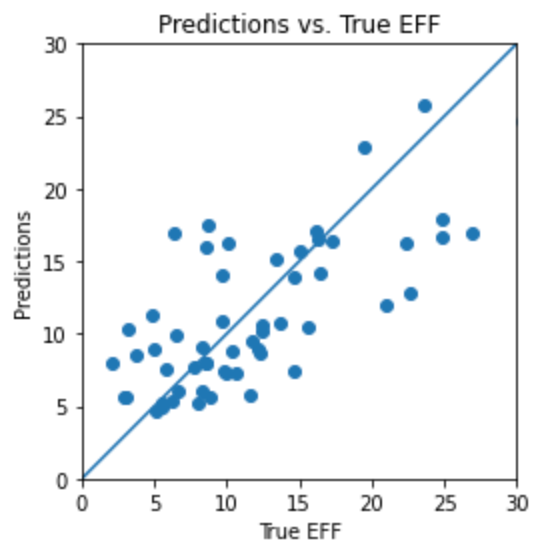
Here is a graph of the XGBoost predictions vs. the true EFF of the injured players after recovery. Even though the dots are somewhat spread out from the diagonal line, we can still see a medium correlation (r^2 = 0.4957) between our prediction and the true EFF, suggesting that our model has captured some of the complexities of the features and is able to use them to predict the EFF of injured players.
Classification Model:
We also wanted to see if we could build a classification model that can tell us whether a player who undergoes an injury will improve or worsen in performance after their injury. The goal this time is to have the model tell us whether Zion’s efficiency rating will increase or decrease after his return. To do this, we used the XGBoost classifier model. Instead of using mean absolute error as the metric, we used accuracy in order to see how well our model does at predicting whether an injured player will increase in efficiency rating. In the end, our model achieved around 72.41% at classifying injured players as to whether they will improve in EFF or not. This seems to be a reasonable result considering that we didn’t have that much training data, and there is still a lot of variability in the player’s performance that simply cannot be predicted by machines.
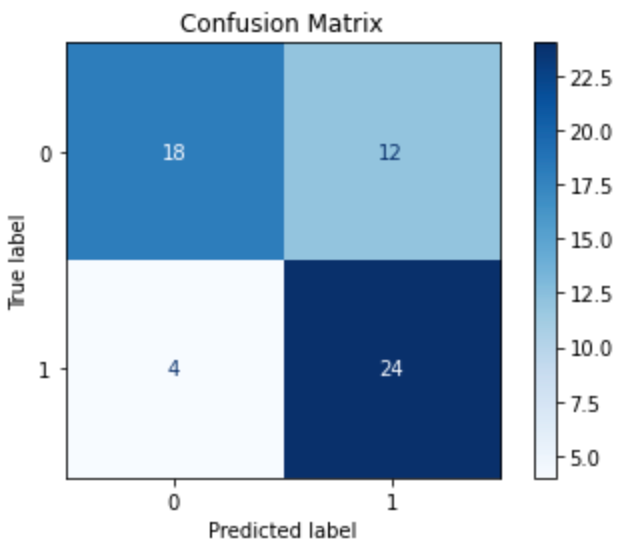
Here is the confusion matrix for our predictions. Notice that the model tends to over predict that the players will increase in EFF. As we will see later on during the case study, this will affect the predictions we get for Zion.
With these models, we are now ready to conduct our case study and predict Zion’s performance on the court after his return this season.
Case Study and Prediction for Zion:
In 2019, Zion Williamson was selected by the Pelicans as the first overall pick. He had an impressive rookie season averaging 22.5 points on 58.3 percent shooting from the floor, 6.3 rebounds, and 2.1 assists per game. However, during the 2021-22 off-season, Williams had suffered a Jones fracture in his right foot and had to undergo surgery. Due to this injury, Williams missed the entire season. But on October 4, 2022, Williamson made his return. There had been lots of speculation about Zion’s long-term availability due to concerns about his size, the nature of his injuries, and the aggressive and explosive ways he plays. The hype surrounding Zion and the striking features affecting his performance renders him a perfect candidate for our analysis.
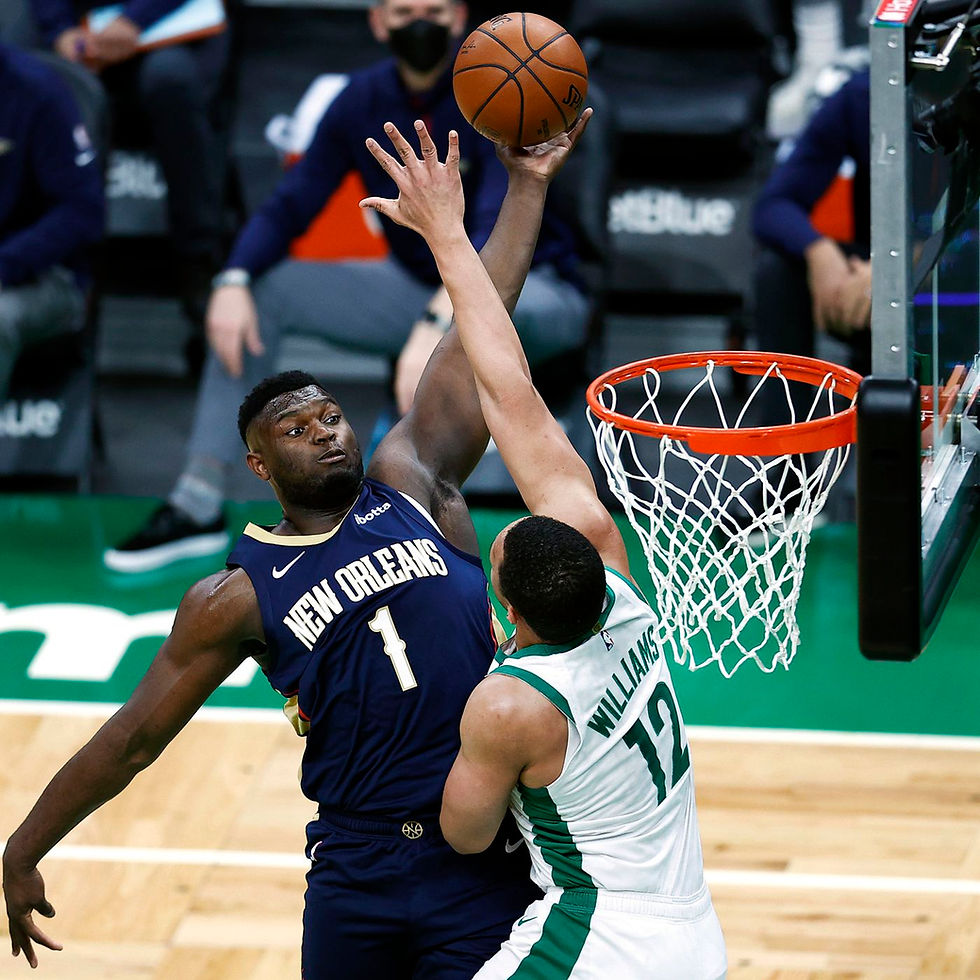
Here is the data that we used to predict Zion’s efficiency after his return:

Height: 200.66cm
Weight: 128.82kg
Age at the time of injury: 20
Previous EFF: 27.4
Injury type: foot
Position: power forward.
Given this information about Zion at the time of his injury, our regression model predicts that his efficiency rating for the current season is going to be about 22.39!

This seems to align with how Zion is performing at the moment, as he has been averaging 24.6 points with 5.4 rebounds and 4.1 assists every night.
We can also strengthen our machine learning model with a somewhat more subjective case analysis. First, except for the weight group, considering age, injury type, and position, we have a young power forward who had experienced foot injury. These features all correspond to more positive comeback performances by our distribution graphs. Regarding the concern of being injury-prone, from online photos, interviews, and team reports we can see the physical transformation, indicating that Zion has taken time to be in the best shape that he can be, in fact losing weight to 284 pounds. The fun fact that he has hired a personal chef also shows that he is committed to staying healthy. However, it is important to note that the explosive and physical way he plays (ups and under, finishing with contact and dunks), still makes him more susceptible to injuries if he does not start to put more shooting and variation into his game.
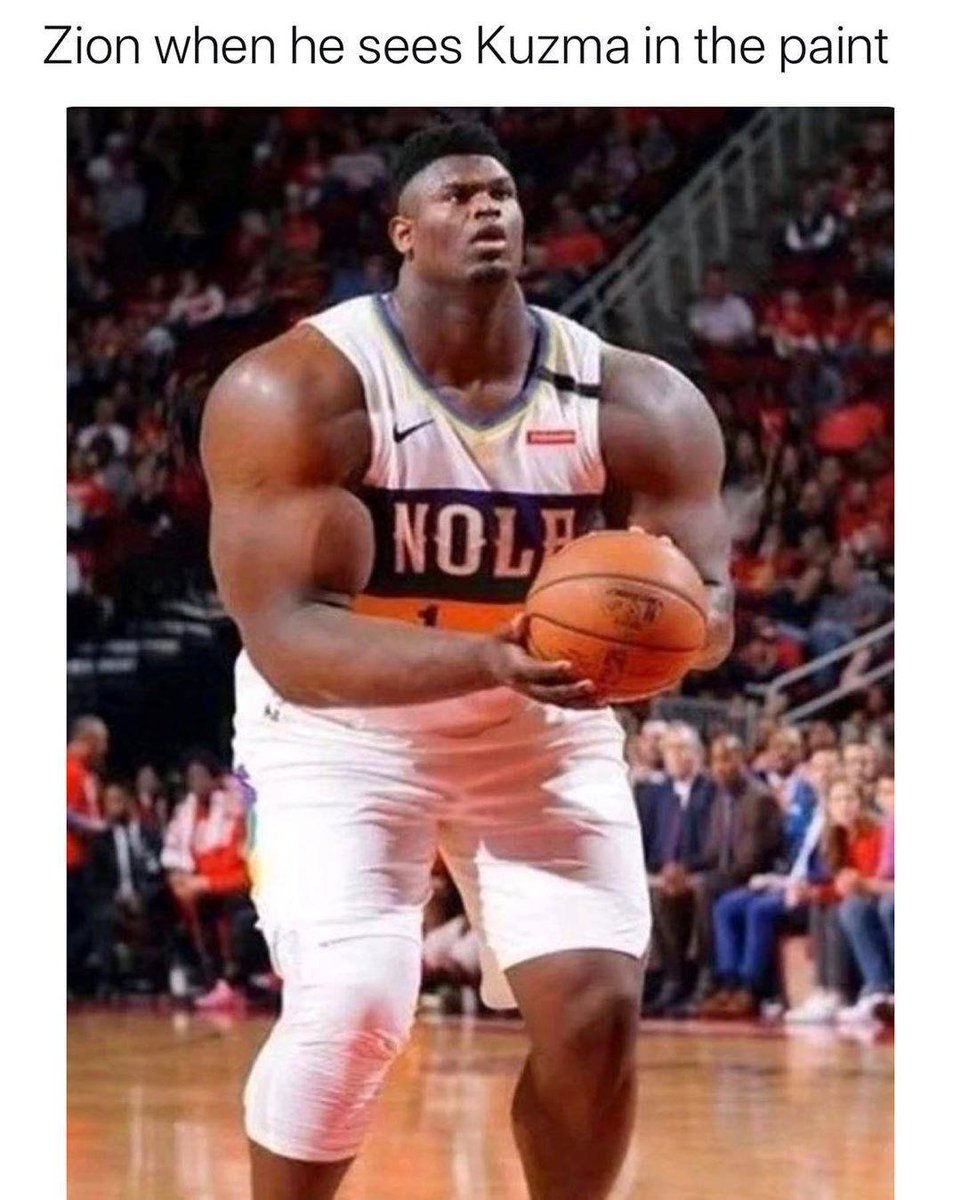
There’s not much concern with game performance as long as a healthy Zion can contest any shot with his freakish athleticism and versatile finishes at the rim. We all know what Zion can do on the offensive end of the floor. However, In terms of field goal attempts, with the trio of Zion Williamson, CJ McCollum, and Brandon Ingram, Zion may not have as many field goal attempts as before as CJ and Brandon both prioritize offense and have relatively high field goal attempts. Thus, we can possibly observe a decrease in average points per game and EFF as a result.
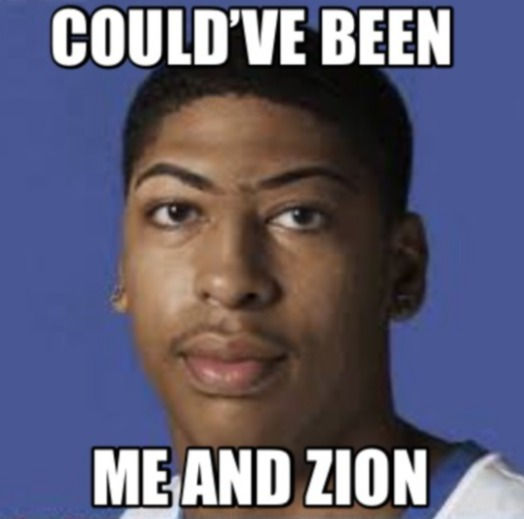
Blake Griffin & Zion Williamson Comparison
We also compared the pre-injury and post-injury data of Zion with Blake Griffin to gain more insights as these two players are both incredibly explosive, athletic, and high-performing Power Forward before the injury.

The fact that Blake Griffin’s EFF decreased by approximately two points provides more confidence and credibility to our model prediction as it aligns with our prediction of Zion’s EFF to decrease.
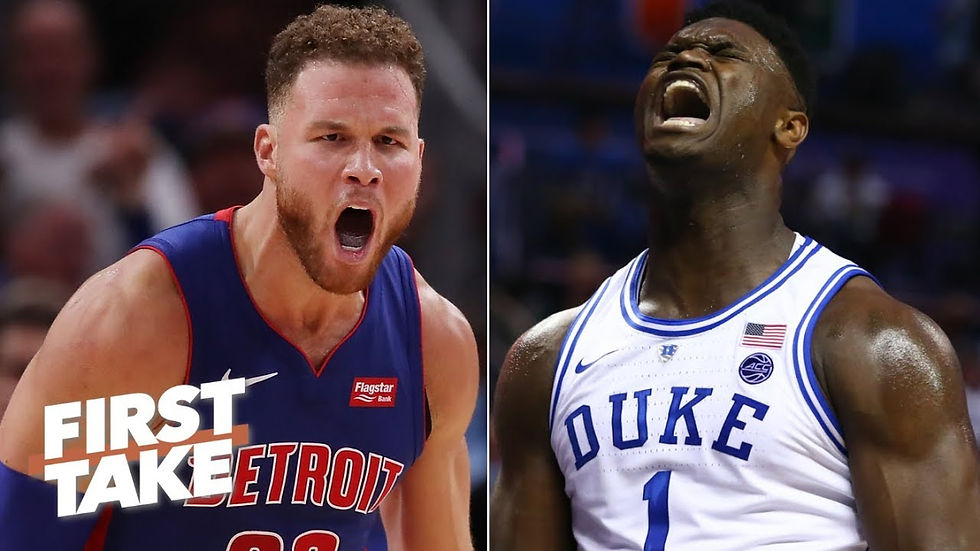
Limitations and Improvement:
Taking account of psychological factors:
One of the important factors that we neglect to address is the psychological factors throughout the return-to-sport process. Studies have shown that players with higher levels of stress, anxiety, and fear of reinjury are less likely to return with higher performance and heal fully. Also, according to numerous interviews, players themselves also perceive coping skills, motivation, and social support as crucial to restoring self-confidence and performing better post-injury. Currently, public datasets online don’t have data that describe psychological attributes and the mindsets of NBA players, nor are these attributes easy to measure on a standardized basis. As for future research, we can try to apply text mining to find more information about players’ psychological status and level of resilience. As a bold suggestion, the NBA league should also take into account the psychological condition of players by having them take outcome measures such as the Resilience Scale for Adults (RSA) that allow not only more statistical analysis but also reference for further psychological assistance.
Post-injury roles and Usage Percentage:
Another important factor that we have neglected to address is Usage Percentage (USG%), which measures the percentage of team plays utilized by a player while they are in the game. The intuition is that when a player suffers from season-ending injuries, the long recovery timespan usually incentivises the team managers to conduct a series of trading and adjustment to having other players substitute their roles on the team. Depending on the performance of other players and many other factors such as the priority and goal of the team, for example, whether the team wants to win a championship or develop rookie talent, returning injured players usually face adjustment to their roles and most of the time decreased roles for offensive gameplays. Therefore, the change in USG% can be a very reliable indicator of how injured players’ roles have changed, which is usually strongly correlated with EFF.
Conclusion
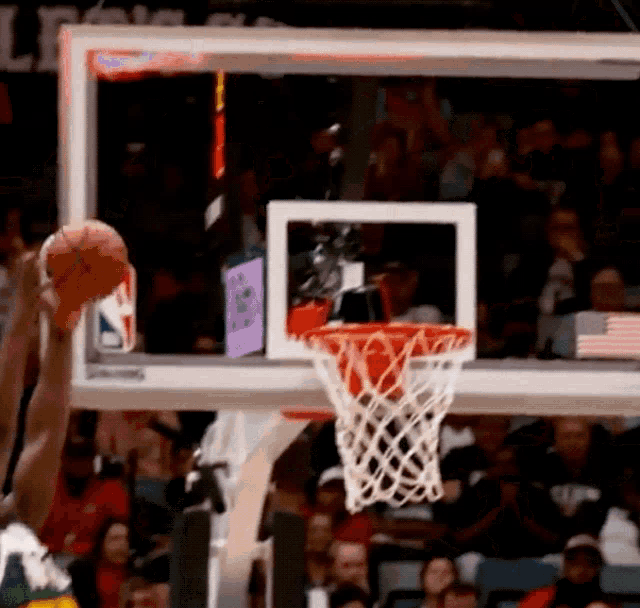
Overall, by our machine learning model and case analysis, we have a high optimism of Zion’s comeback during the 2022-2023 NBA season. Speaking of analytical patterns, most players with the injury-related attributes tend to have successful comebacks. Considering that he is a young super talent and making an effort to control his weight and physique, and the fact that the Pelicans are cautious with his minutes on the floor, we are sure that Zion will still play All-Star level of basketball. However, this does not mean that Zion’s EFF will increase. In fact, our XGBoost model and regression model both predict that the EFF will decrease. This prediction is reasonable due to concern with his previous weight, explosive and risky playing style, and the decrease with USG% as CJ McCollum joins the squad. Lastly, let's end the article with our favorite quote by Zion, and by wishing “good luck” to the other teams, because Zion is going to be unstoppable.
"I just look to be myself. I'm not trying to be nobody. I'm just trying to be the first Zion." - Zion Williamson
Sources:
Comments